
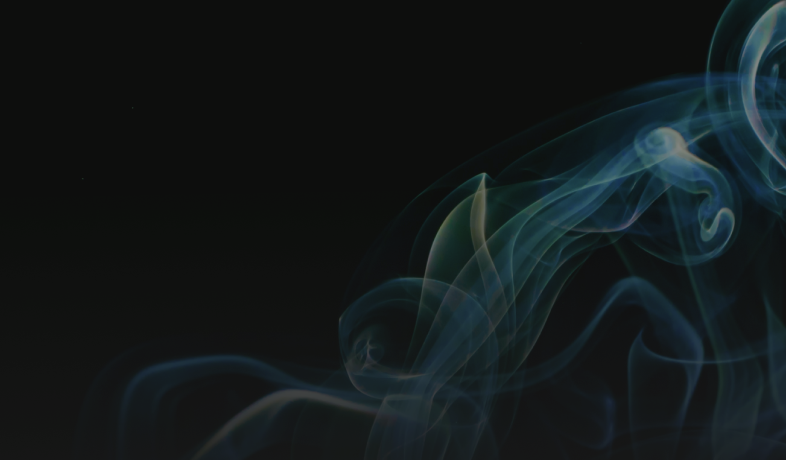
Metagenomic Data Analysis on Steroids: Insights from Dr. Martin Steinegger
Lifebit
Preface
Our new series entitled “Bioinformatics Superheroes”, aims to focus the spotlight on researchers that have heavily contributed to the field of bioinformatics, in any way, shape or form. Importantly, through this series, we delve into the main challenges in today’s world of bioinformatics and learn about these from the experts themselves. We hope that this new series will give readers a different perspective of issues at hand, which might, in turn, be a call to action to encourage finding solutions to these problems.
For more information on what inspired this series please a look at this blog post, where CEO Dr. Maria Chatzou unveils the challenges that she faced as a bioinformatician, and how she solved these through the development of Lifebit’s platform, CloudOS.
We start our series with Dr. Martin Steinegger who has always been obsessed with: how can we get insights from analysing massive amounts of metagenomics data in only minutes, instead of the days and weeks that the process currently takes. Or in other words, how to perform metagenomics analysis on steroids. This most likely stems from the fact that Martin is of an impatient nature, and he actively works to increase the efficiency of methods to get to tangible results faster, thereby facilitating his own work.
During his PhD, Martin dedicated the bulk of his time to developing his method trilogy (MMSeqs2, Linclust, and Plass) which is aimed at facilitating metagenomic data analysis – (published in Nature Biotechnology and Nature Communications, congrats!).
The Problem
To start off, what is metagenomics? Metagenomics is an approach to understand the genetic composition of a community, thereby transcending the individual to focus on the whole. Communities in this context refers to microbial communities that coexist within organisms (i.e. humans), or within environmental samples (i.e. soil, water). This seriously complicates analytical methods as you are not dealing with only one genome but with a collection of hundreds to millions of bacterial genomes that serve collective functions.
Until recently, microbes were purified from a sample and sequenced separately in order to simplify genome assembly (imagine having to assemble a genome with ‘contaminating’ fragments from other similar microbial genomes floating around). However, by simplifying assembly, we were also losing the microbial diversity as most microbes do not fare well in a petri dish. A better alternative, that allows scientists to capture the whole microbial population is metagenomic analysis, which detects all the strains present in a sample, even novel strains that have not yet been described.
Now that sequencing prices are much less prohibitive than they used to be, metagenomics is booming and is being applied to many different fields (earth and life sciences, bioremediation, biomedical sciences, agriculture, hygiene, cosmetics and nutrition, among others). However, it’s not all fun and games. For Martin, the biggest challenge in metagenomic analysis is the diversity issue.
“There is so much diversity in these samples. Strains are not just one genome, they are very closely related and they accumulate all kinds of mutations…you have a gradient of mutations.”
Our Superhero & his solution
To tackle diversity, Martin based his tools on the protein level instead of the nucleotide level, because on the nucleotide level “the synonymous SNPs disappear as they encode for the same amino acids. Proteins, on the other hand, have a huge selective pressure, so you cannot randomly mutate them”.
Martin admits that he actually developed his open-source method trilogy upside down: Plass → Linclust → MMseqs2. When you acquire the sequencing reads from your human or environmental sample of interest, you feed them into Plass (Protein-Level ASSembler), which is a de novo tool that assembles protein sequencing reads into protein sequences. Plass comes with a redundancy-filtered reference protein catalogues which covers two billion sequences from soil and marine samples – the largest free collections of protein sequences1. Plass will output your protein sequences which are “often highly redundant because you assemble all kinds of combinations from the same strain” says Martin. Once Plass has digested your raw data, you will be left with about 100-200 GB of protein data that you somehow have to reduce.
That’s where Linclust comes in. Linclust is a fast clustering algorithm that can cluster large datasets. To give an example, it can cluster 1.6 billion metagenomic sequences down to 50% sequence identity in 10h on a single server – more than 1000x times faster than has ever been possible2. That’s quite a few zeros there!
Once, you have reduced the redundancy of your protein sequence set with Linclust, you can use MMseqs2 (Many-against-Many sequence searching 2) for functional annotation. You take all your protein sequences and search them against the current databases to try and figure out which pathways and functions might exist. You can also use MMseqs2 to map back the initial DNA reads to perform abundance analysis in order to know the quantities of a certain strain in your sample.
Martin has gotten a lot of positive response from his tools, indicating that there is a huge need for them in the field: “you can see researchers starting to explore how to use the tools in their pipelines”. Martin notes that clustering is of significant importance to researchers because the amount of data is accumulating so fast, and linear time helps by speeding up the process and not having to wait for weeks to get results. He develops his tools with PhD students in mind, to hopefully improve their quality of life and save their time while also enabling novel large analyses which provide insights on our environment.
For Martin, developing bioinformatic methods means “to be able to understand the underlying information and how to maximise the extracted information efficiently”. For instance, the evolution of homology search methods starting off with sequence vs. sequence searches < profile vs. sequences < profile-HMM vs sequence < profile-HMM vs. profile-HMM (< means uses less information than). In this case, the information for profile-HMM searches was always there, but it just took a lot of time to leverage it.
What is next for our Superhero?
Martin is looking to expand his tool offering to combine the nucleotide and protein worlds. He has always felt like protein and nucleotides are treated separately: people tend to either look at proteins or at nucleotides. According to Martin “combining both worlds can be valuable and complementary and when it comes to assembly, you can improve sensitivity and specificity at the same time”. And we’re eager to see your new work, Martin! No pressure…
Martin is excited to be part of the technological innovation, which transforms the way we do biology. The rapid development of sequencing technologies means that we are currently in the midst of a huge data explosion, which for Martin “is incredible fun to tackle issues associated to the data overload”.
About Dr. Martin Steinegger
Although Martin has a strong computational background having worked as a software developer in various companies, he decided to complete his B.Sc. in Bioinformatics at the TUM/LMU Munich because he wanted to learn more about biology. However, after finishing his bachelors, Martin decided to be more practical and do his Masters in Computer Science because he was tired of biochemistry and memorising so much information. Who can blame him?
As he embarked on his PhD journey, his supervisor gave him the opportunity to deepen his knowledge and work from top laboratories around the world, including: Dr. Andrej Sali at UCSF (San Francisco), Dr. Cedric Notredame at CRG (Barcelona) and Dr. Chaok Seok at Seoul National University (Seoul). This flexibility gave him a chance to see similar problems from different perspectives.
Martin has earned his PhD in Computer Science at the Technical University of Munich, working under the guidance of Dr. Johannes Söding at the Max Planck Institute for Biophysical Chemistry in Göttingen. Recently, he has started his postdoc in Dr. Steven Salzberg’s Computational Biology and Genomics laboratory at John Hopkins University.
Congrats on the new position & good luck with everything, Dr. Steinegger!
References
- Steinegger, Martin, Milot Mirdita, and Johannes Soding. “Protein-level assembly increases protein sequence recovery from metagenomic samples manyfold.” bioRxiv (2018): 386110.
- Steinegger, Martin, and Johannes Söding. “Clustering huge protein sequence sets in linear time.” Nature communications 9.1 (2018): 2542.
- Steinegger, Martin, and Johannes Söding. “MMseqs2 enables sensitive protein sequence searching for the analysis of massive data sets.” Nature biotechnology 35.11 (2017): 1026.
We would like to know what you think! Please fill out the following form or contact us at hello@lifebit.ai. We welcome your comments and suggestions!
Featured news and events
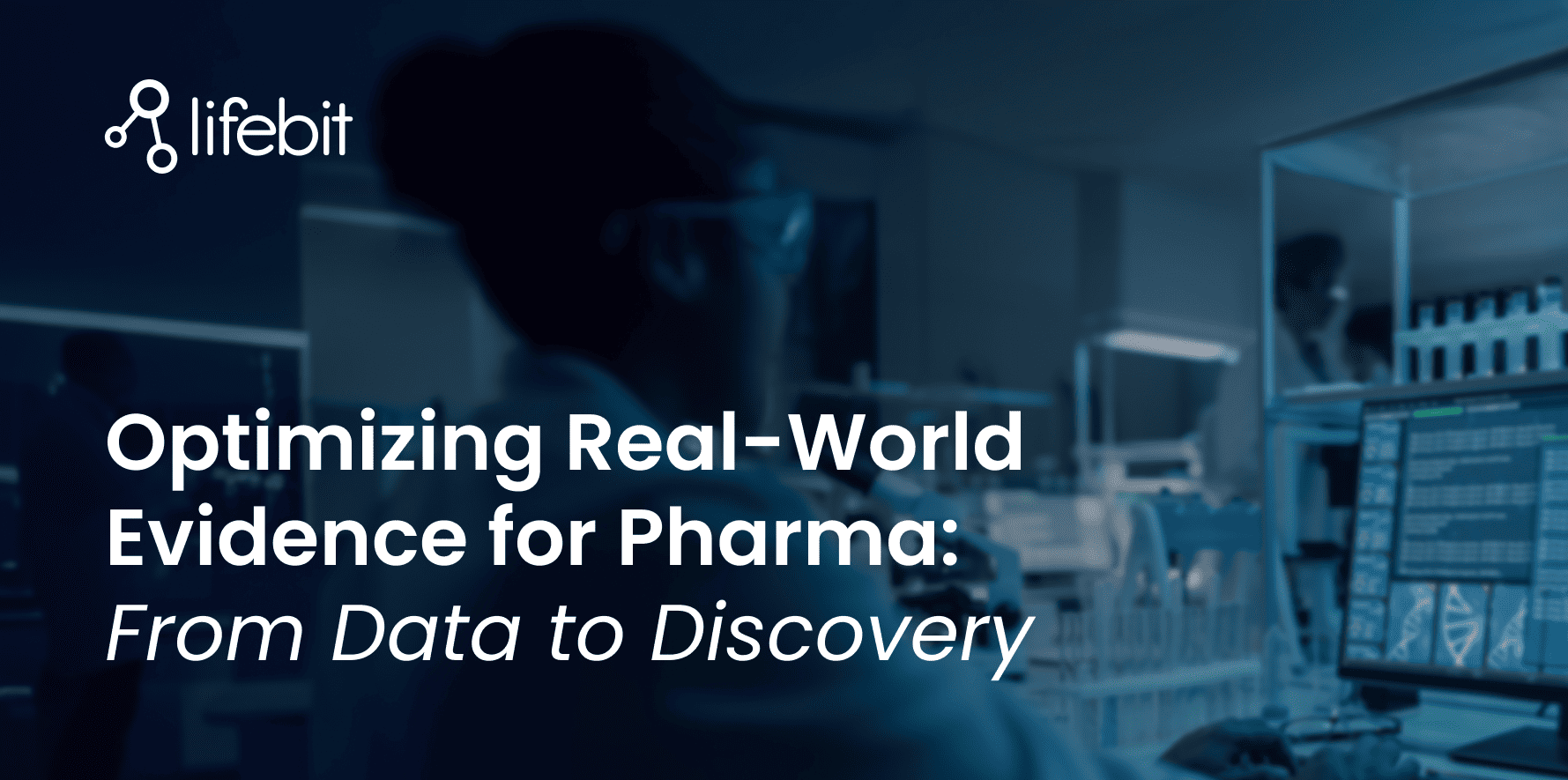
2025-02-19 13:30:24
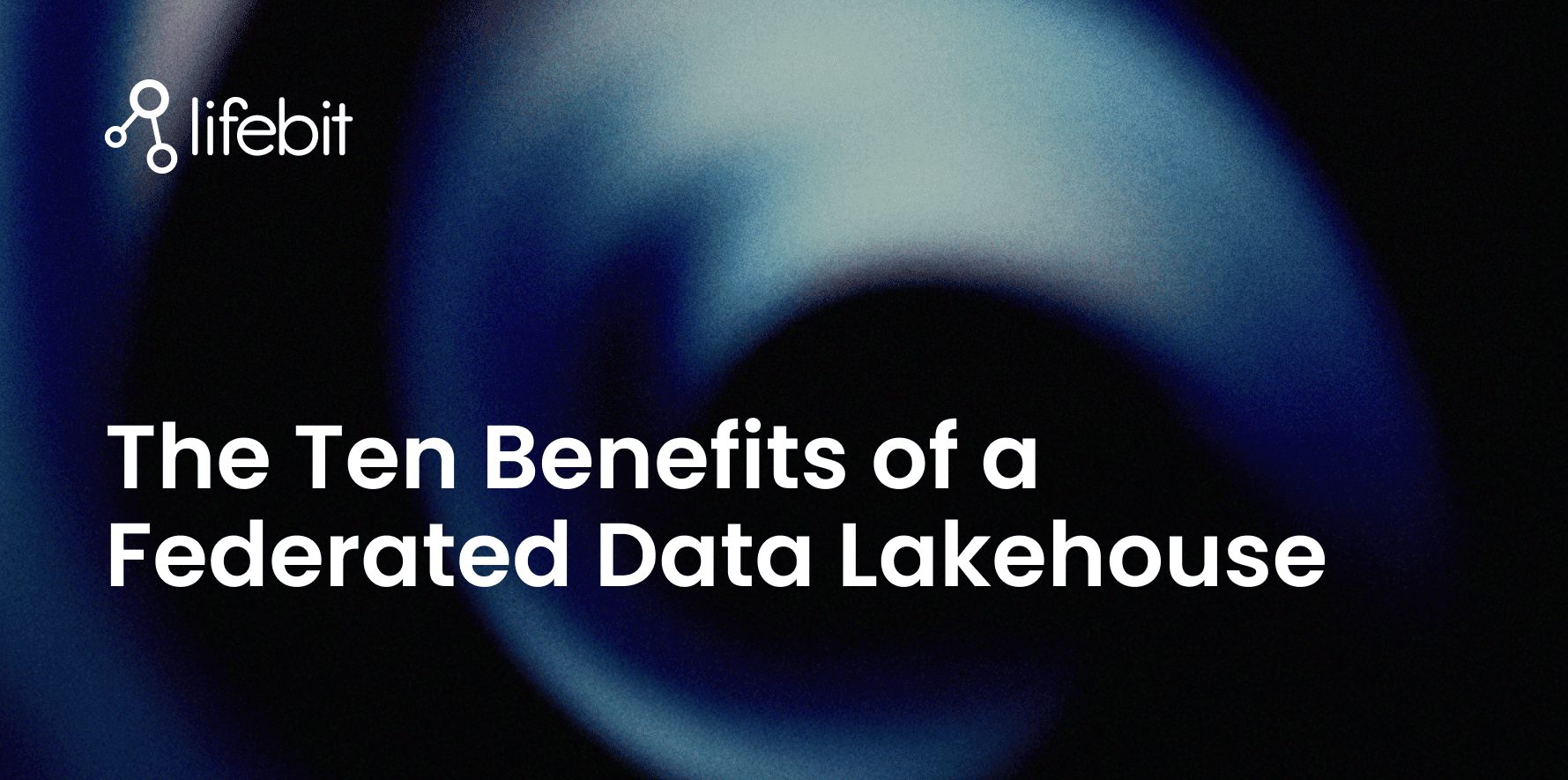
2025-01-30 12:47:38
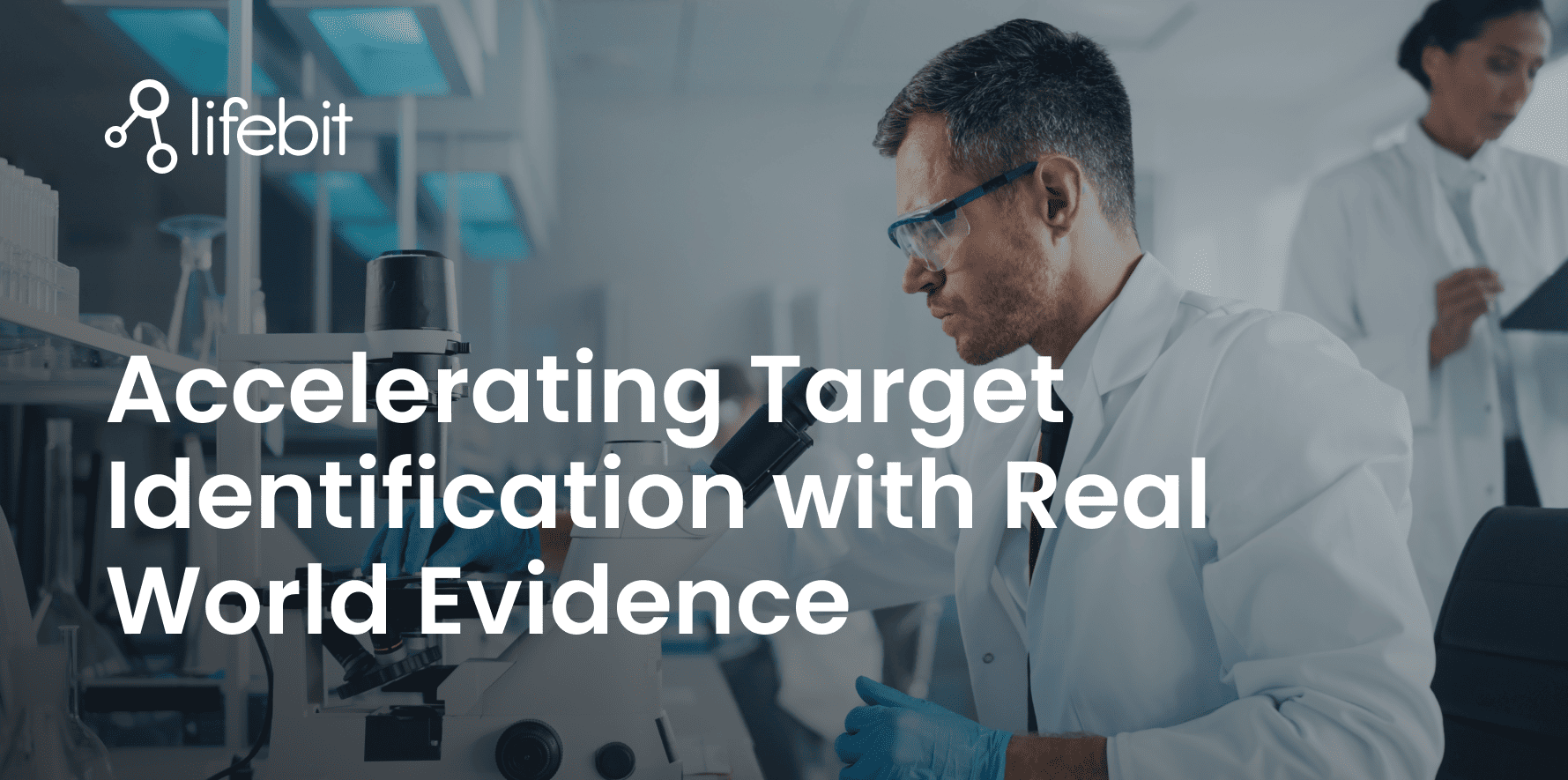
2024-12-06 15:53:10
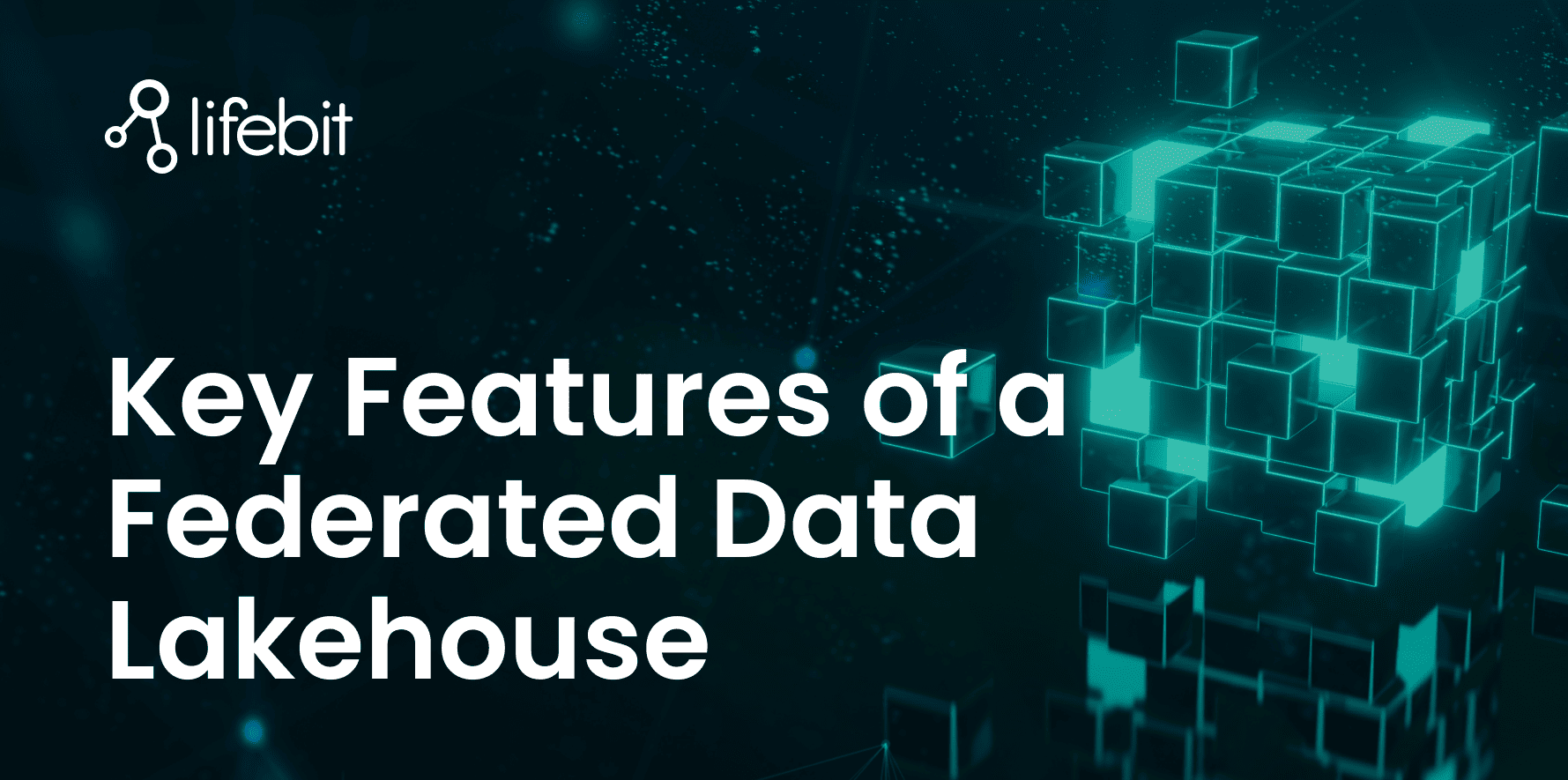
2024-11-27 12:35:34
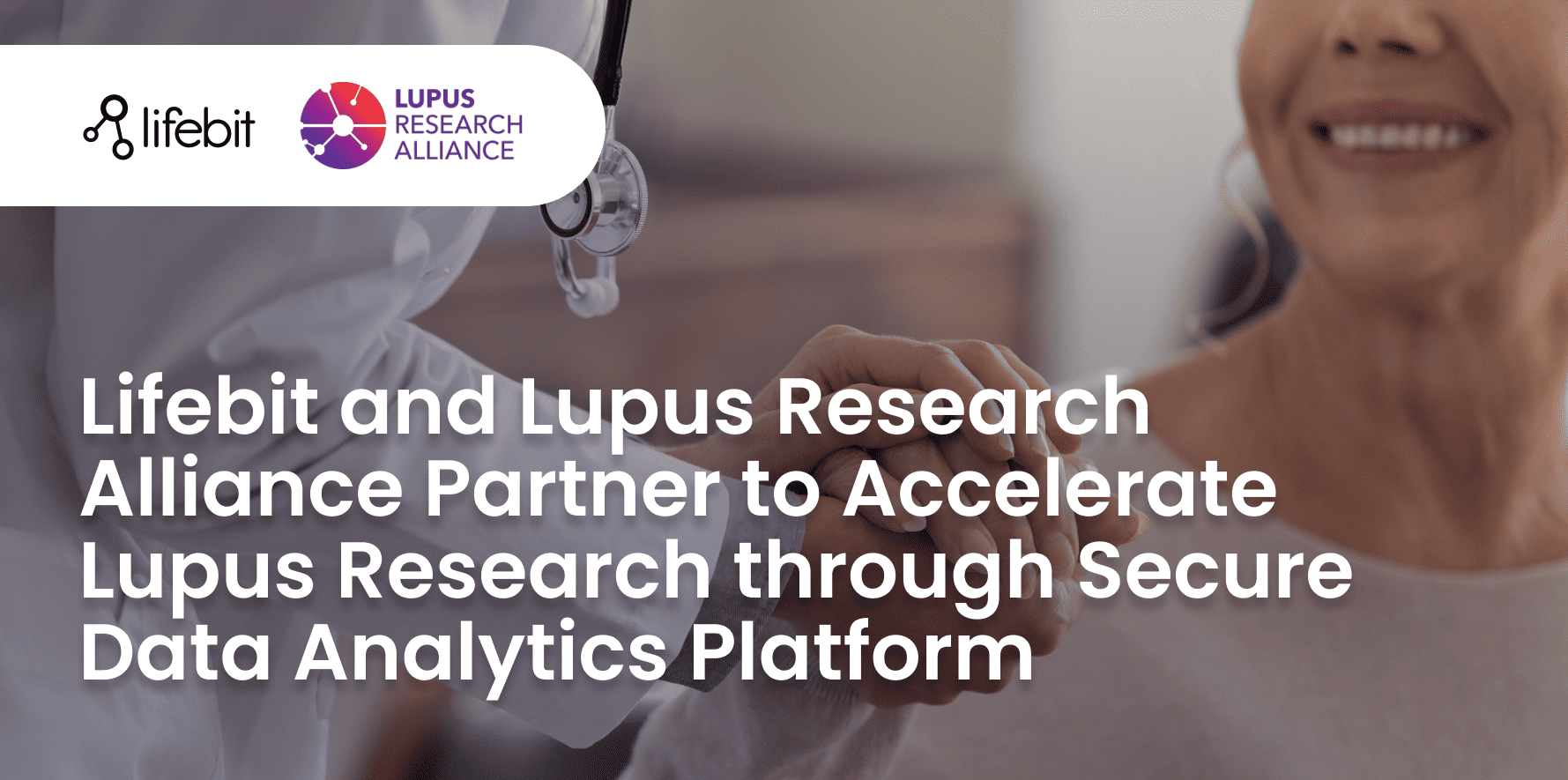
2024-11-27 09:15:04
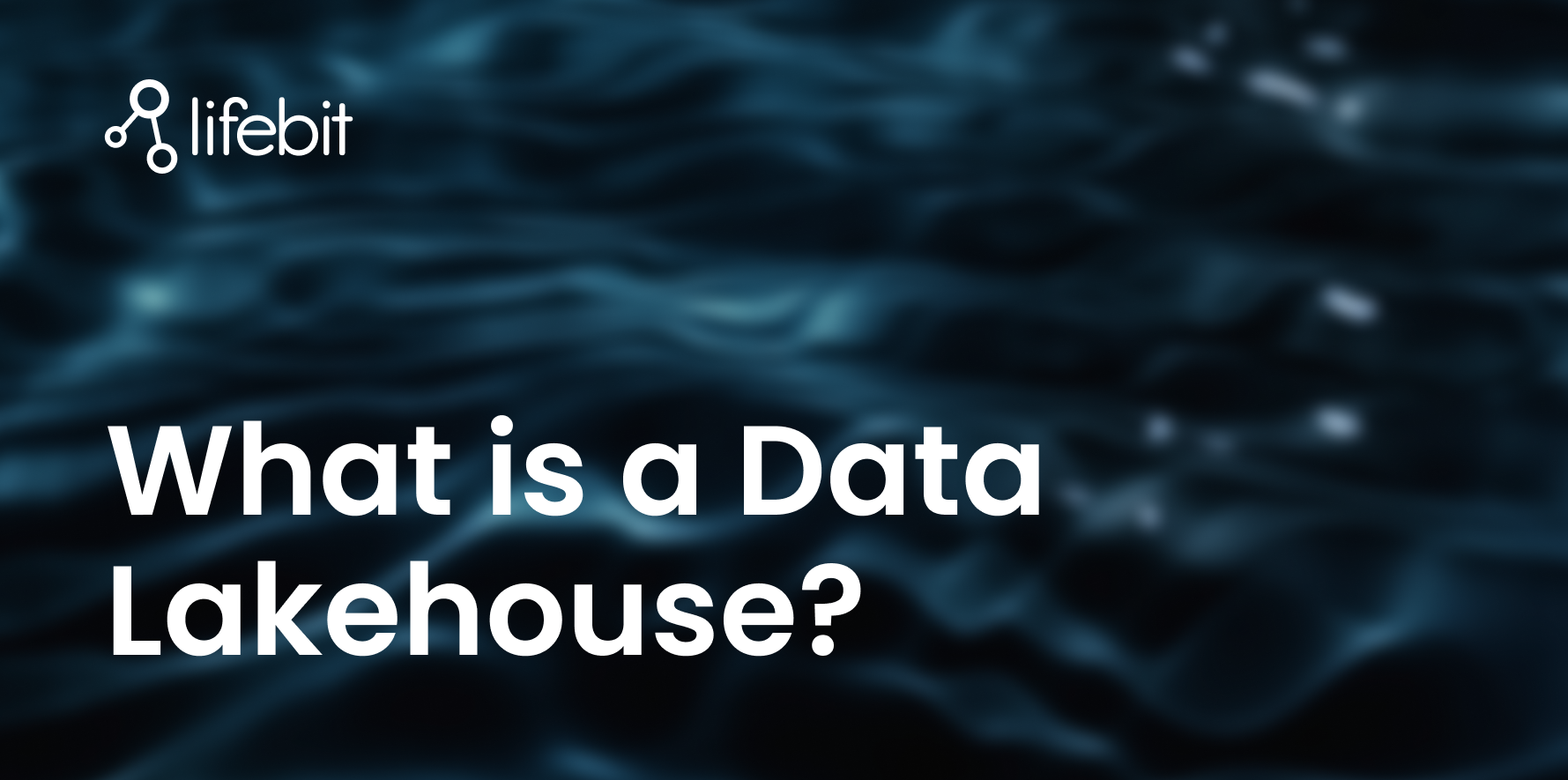
2024-11-20 09:41:45