
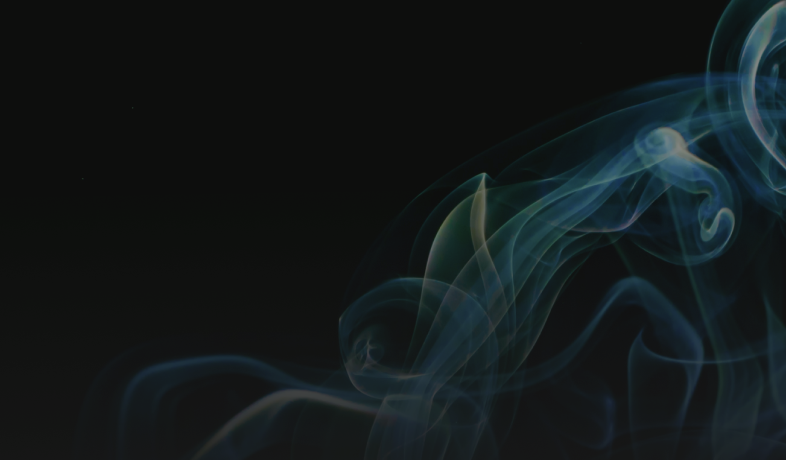
Leveraging AI for target validation in drug discovery
Lifebit
Introduction to health data, drug discovery and AI
Healthcare is one of the most data-rich industries and the creation of large datasets is becoming increasingly common due to developments in technology, data science and new skills. These technologies include lower cost genome sequencing, the digitization of medical technologies, and the accumulation of electronic health records (EHRs).
The vast health data created from millions of patient interactions with health and life sciences sectors across the world is increasingly used in research to power studies such as population genomics initiatives and to identify and validate new therapies in the drug discovery process. This is evident from the increasing number of search results on PubMed for 'health data', as outlined in the graph below.
Health data is needed to develop new disease treatments in drug discovery. However, due to the volume of data that needs to be analyzed, researchers can struggle to utilize this data effectively.
Artificial intelligence (AI), a field that uses robust datasets and computer science to facilitate problem-solving, can help researchers stay ahead of the curve. This article discusses the ways AI is accelerating the drug discovery process.
The process of drug discovery
Target identification, or understanding what process or molecule (e.g. RNA, protein or a specific cellular pathway) a medicine should target to prevent disease progression, is the first step in the drug discovery process. The next step involves the experimental validation of a target, known as target validation. Once this is achieved, drug candidates can move into pre-clinical development before moving on to clinical development and patient testing.
The crucial role of AI in target validation
Historically, finding a drug candidate has required laborious and complex screenings to find possible chemicals in a library that have the desired effect.
-1.png?width=41&height=60&name=Vector%20(1)-1.png)
-1.png?width=41&height=60&name=Vector%20(1)-1.png)
However, more efficient target validation might cut phase II clinical trial attrition by around 24%, saving the cost of creating new drugs by roughly 30%.
-1.png?width=41&height=61&name=Vector%20(2)-1.png)
-1.png?width=41&height=61&name=Vector%20(2)-1.png)
Developing a more robust understanding of biology is the first step in finding more suitable drug targets. This is increasingly derived from genomic insights, which uncover the genetic changes underlying disease using large-scale health data from patients and national biobanks.
Featured resource: Discover our whitepaper on the future of genomic data management
With recent progress in genomics and AI, researchers are improving target validation by concentrating on identifying original and innovative drug targets.
AI is helpful in drug discovery in three key areas:
1. To find possible targets that are involved in disease processes, machine learning (ML) algorithms can analyze a variety of datasets, such as gene expression profiles, protein-protein interaction networks, and genomic and proteomic data.
2. AI can precisely predict a possible compound's physical and chemical characteristics. These characteristics, including the drug's solubility, partition coefficient (logP), degree of ionization, and intrinsic permeability, must be considered because they indirectly impact the drug's activity. The advantages are apparent here: neither money nor effort is wasted on substances unlikely to produce the intended results.
3. AI can aid in synthesizing new compounds by generating molecules with characteristics that are expected to be successful. This could significantly enhance the finding and production of novel, potent medications. Furthermore, when determining a drug's efficacy, repetitive work can be eliminated by using AI in this process.
An example of AI in action in drug discovery
Recently, a novel A2 receptor antagonist intended to support T cells in attacking cancer has entered human clinical trials. The A2 receptor antagonist, which is in development for adult patients with advanced solid tumors, was developed with the aid of AI.
An AI drug discovery programme that can computationally sort, compare, and rate a large number of compounds was used in this study. This method selected clinical trial candidates from millions of starter molecules. This could potentially lead to the development of an intriguing new cancer treatment.
Future outlook: drug discovery 2.0
Drug development will radically change due to improved target discovery and validation by integrating large scale health care data, genomics, and AI. Fully utilizing the large-scale health and genomic data collected will be crucial in obtaining valuable insights, developing novel and effective drugs and enhancing patient outcomes.
Summary
Better access, integration and analysis of health and disease data can help develop new drugs. This will enhance diagnosis, treatment, and prevention strategies, ultimately improving patient outcomes.
AI can help in these endeavors, effectively as a tool for integrating and analyzing these vast and complex datasets. However, there are still challenges to overcome in using AI to solve these challenges- look out for our next blog, which will consider the issues and the solutions being developed to ensure we can get the most out of AI.
About Lifebit
Lifebit provides health data solutions for clients, including Genomics England, Boehringer Ingelheim, Flatiron Health and more, to help researchers transform data into discoveries.
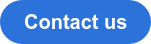
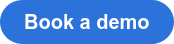
Featured news and events

2025-01-08 13:58:41
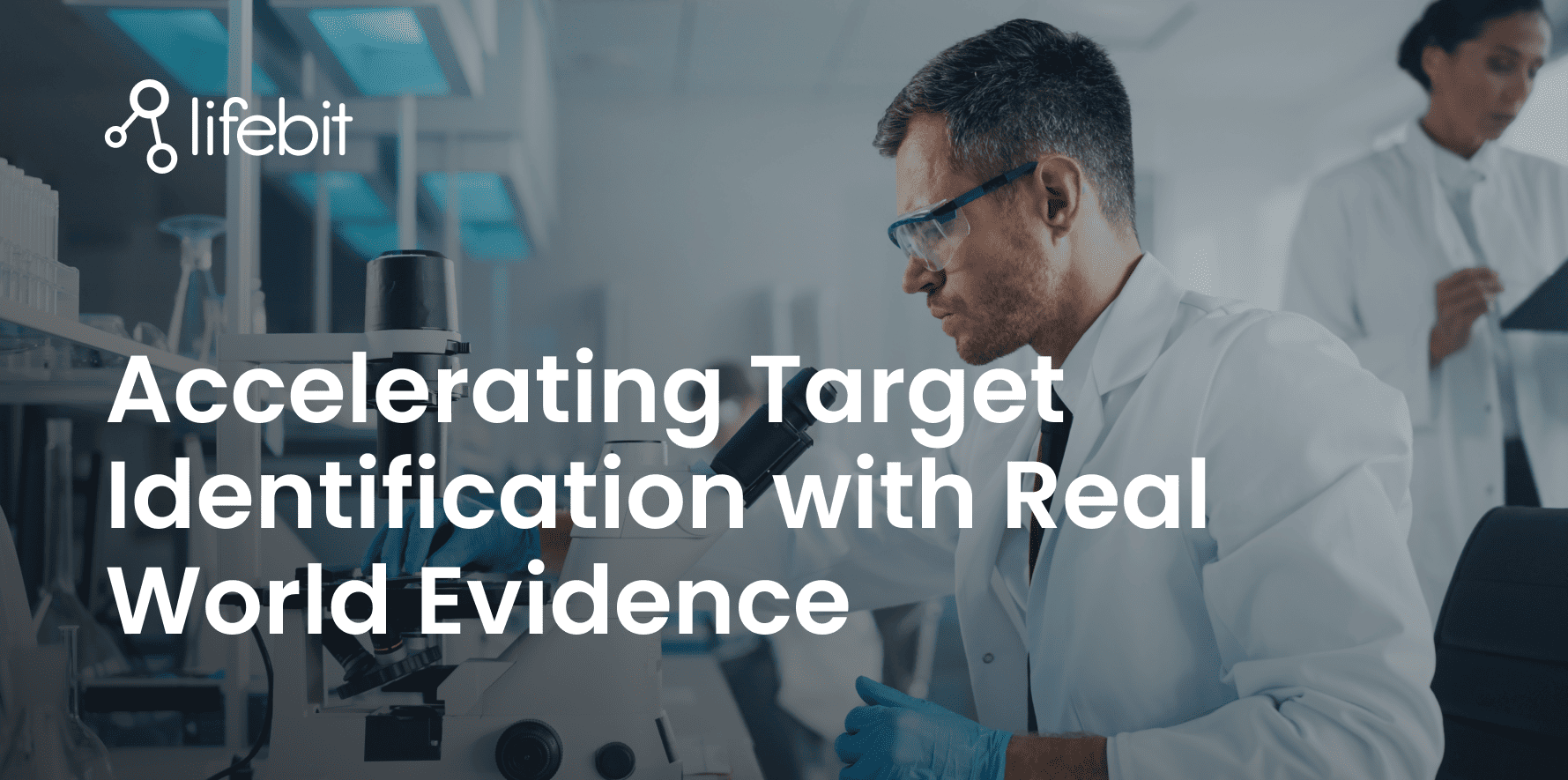
2024-12-06 15:53:10
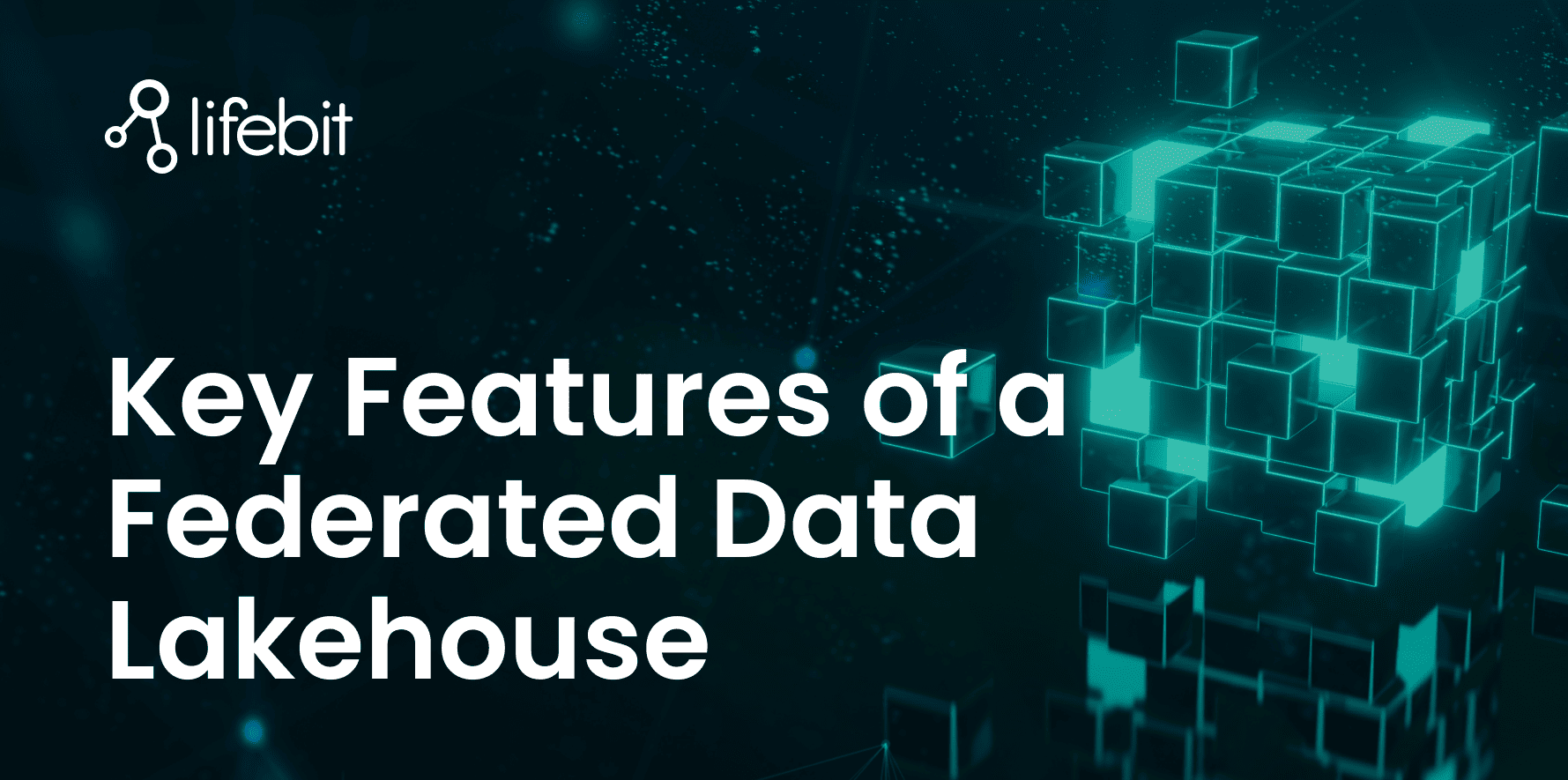
2024-11-27 12:35:34
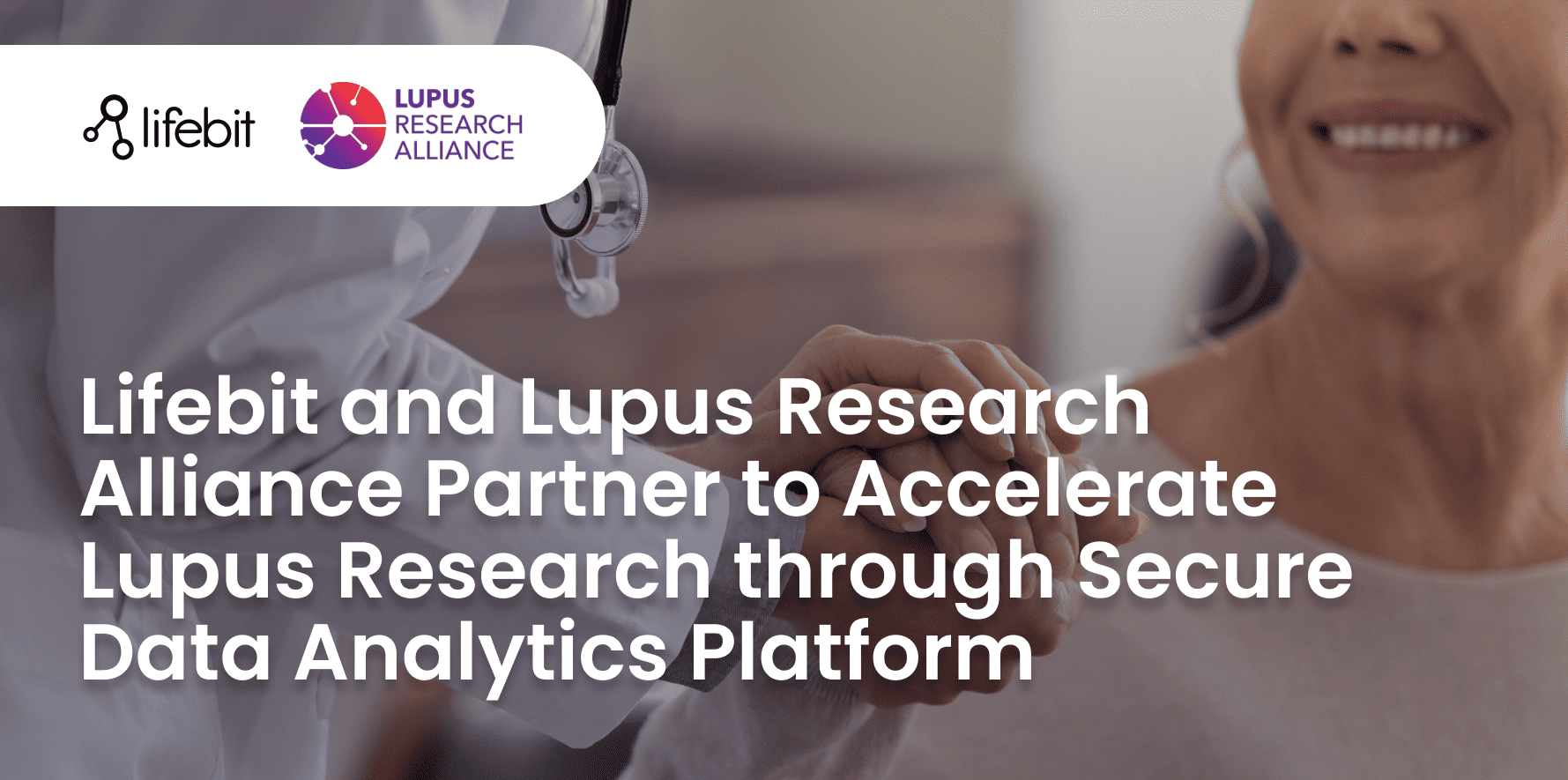
2024-11-27 09:15:04
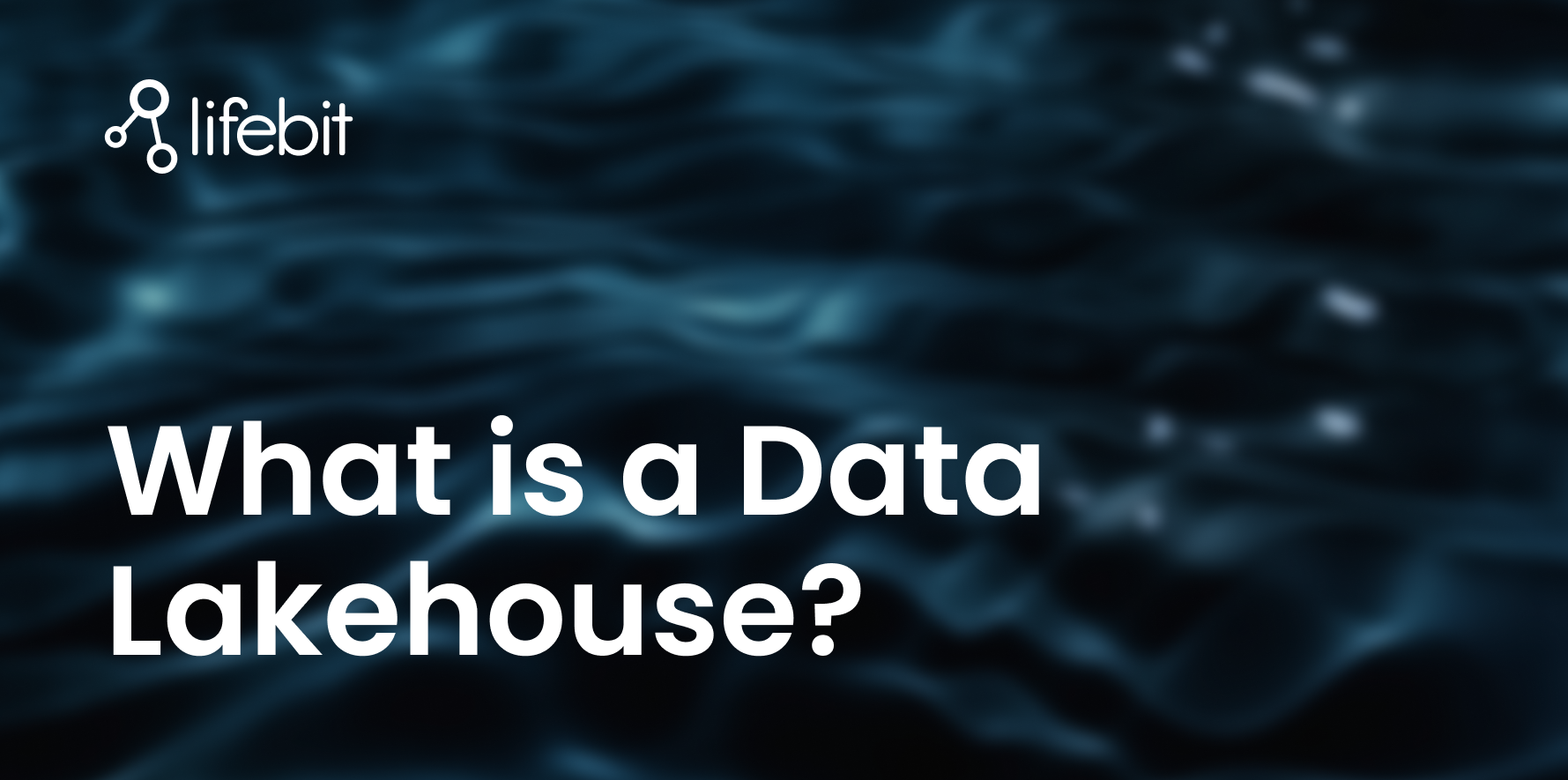
2024-11-20 09:41:45
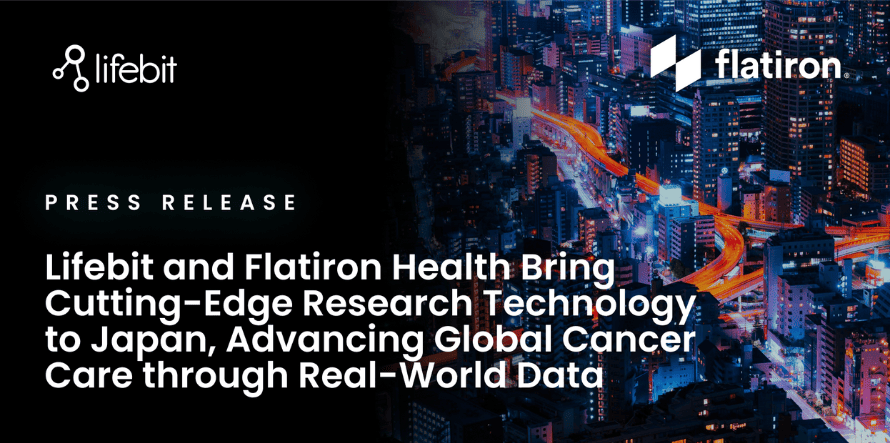
2024-10-08 13:00:00
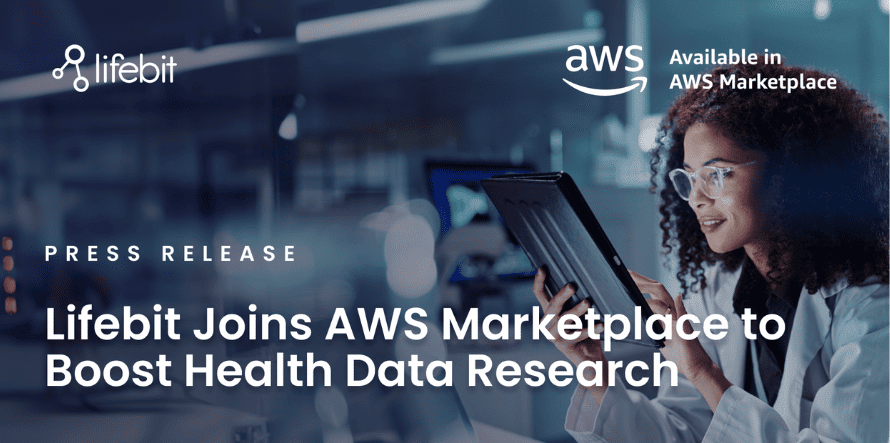
2024-10-04 12:00:00
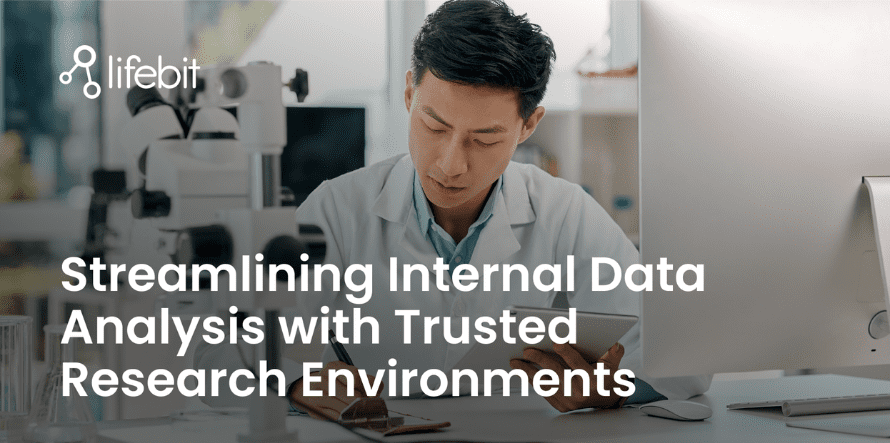
2024-09-24 08:28:11
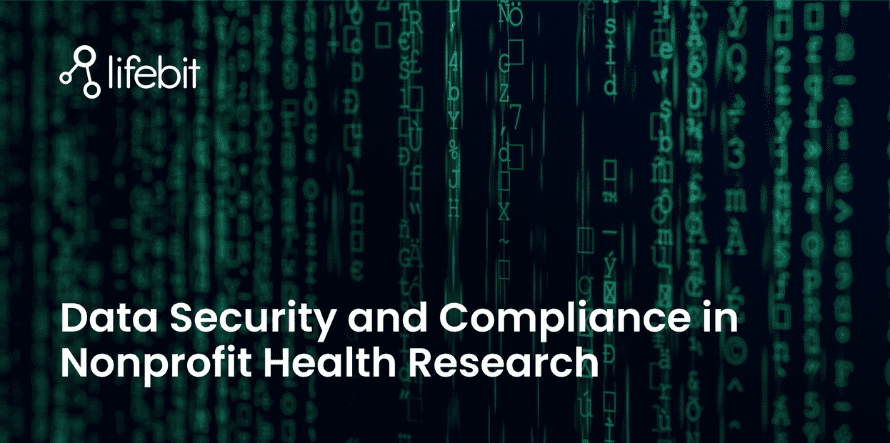
2024-09-19 11:24:21
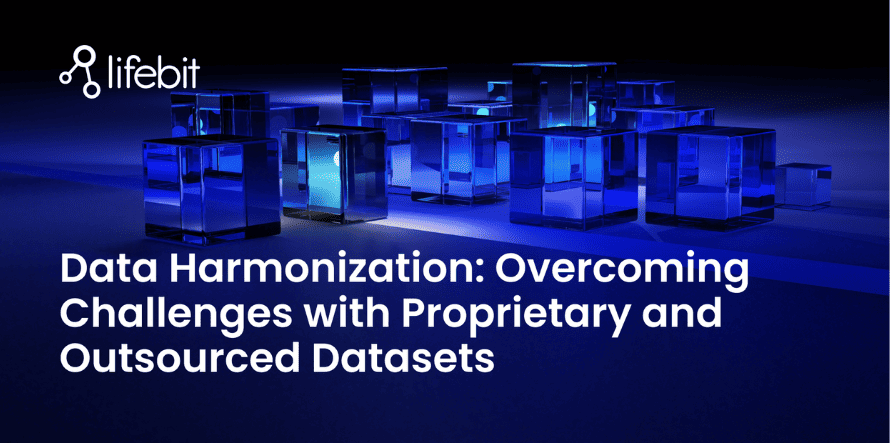
2024-09-18 11:30:00