
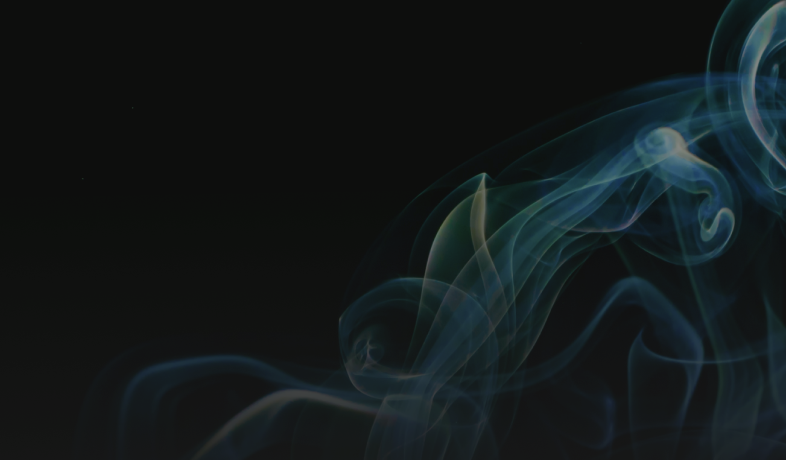
What are the current challenges of drug discovery?
Maria Alvarellos
Introduction - drug development requires time and money
New drugs must meet very high efficacy and safety standards in large, randomized controlled clinical trials (RCT) before they can be brought to market. This helps explain why drug development is a costly and lengthy enterprise: the average time to get a drug to market is between 10 to 15 years, with an average cost of $2 billion. Another study estimated that pharmaceutical companies spend $6 billion per new drug despite a 6% annual increase in R&D over the last 20 years.
This blog post will review the current drug discovery paradigm and its challenges and how new computational tools can help overcome those challenges.
Drug development is a long and meticulous process
Before a drug reaches clinical trials, researchers evaluate it in cellular systems and animals. They first identify and validate 'targets' (often proteins) predicted to cause a disease that candidate small molecules, proteins, or monoclonal antibodies can modulate. This process is generally termed ‘drug discovery’
(Figure 1). The basic steps include:
Figure 1. The process of drug development.
-
Target identification involves identifying a specific biological molecule or pathway (e.g., a protein, enzyme, or receptor) associated with a particular disease or condition that appears to play a vital role in the disease's development or progression.
-
Target validation confirms that a biological molecule or pathway is involved in a disease process and that modifying its activity will have the desired therapeutic effect.
-
'Hit' identification is a stage where high throughput screens (HTS) identify molecules that interact with the target in non-cellular, biophysical assays - these are termed 'hit' molecules because they demonstrate specific activity at the target.
-
Lead discovery is where hits are screened in cell-based assays predictive of the disease state and animal disease models to characterize their efficacy and safety profile.
-
Hit-to-lead (H2L) is a stage where researchers identify their preferred hit series by evaluating potency, selectivity, solubility, permeability, metabolic stability, and pharmacokinetics (PK) in animal models.
-
Lead optimization is when promising lead candidate molecules are optimized by modifying their chemical structure.
The challenges of drug discovery 1.0
If a drug does make it to clinical trials, it is highly likely to fail: only 4% of drug development programs result in licensed drugs. For biologics (including protein-based drugs, monoclonal antibodies, and vaccines), which are taking an increasingly larger share of the market, fewer than 10% succeed in clinical trials with costs estimated to be between $30 and $310 million per trial.
The principal cause of failure is a lack of demonstrable efficacy and is attributed to early missteps during target identification or validation. Why?
-
Preclinical experiments in cells, tissues, and animal models are imperfect representations of human disease, and positive results in model systems or organisms may not replicate in human participants.
-
Small sample sizes in these experiments may also lead to false positives, and only when these false leads are evaluated in costly clinical trials is their lack of efficacy confirmed.
Only 4% of drug development programs result in licensed drugs.
These errors reflect an insufficient understanding of the proposed biological model of disease, which can also result in patients experiencing unexpected and intolerable adverse effects in clinical trials and early termination of drug development programs.
The impact on healthcare systems and patients
As explained above, the inflated price of many prescription drugs reflects inefficiencies in drug development pipelines. The impacts of such inefficiencies include:
-
Increased prices of prescription drugs for healthcare systems, payers, and out-of-pocket costs for patients, sometimes making them wholly unaffordable and inaccessible.
-
Pharmaceutical companies are less inclined to work on more innovative therapeutics where the pathology is more uncertain in favor of new formulations of existing drugs. This results in a handful of chronic diseases with multiple treatments, while many diseases remain without effective treatments.
-
Neglected tropical diseases (NTDs), which, as their name suggests, are often overlooked because of a lack of financial incentives to invest in R&D for new treatments that will be primarily used in developing countries where medicine prices must be kept low.
Drug development moves towards the future - drug discovery 2.0
Experts in the field suggest that one way to improve productivity in R&D is to decrease the attrition of drug candidates at each stage of drug development, beginning with drug discovery. Lower costs for high-throughput sequencing technologies (e.g., whole-genome sequencing), digitization of health records, and increased computing power have led to massive increases in biomedical data. These developments have poised artificial intelligence (AI) and machine learning (ML) as the most likely solutions to make sense of such large datasets to accelerate drug development via data-driven drug discovery (aka Drug Discovery 2.0).
AI and ML tools can rapidly identify novel compounds and targets to speed up drug discovery... In one striking example...the total time from project launch to preclinical testing was four months.
AI and ML tools can rapidly identify novel compounds and targets to speed up drug discovery. Furthermore, even when experimental results are negative, negative results feedback can enhance future prediction models. In one striking example, AI-assisted drug discovery identified nine small molecules from a compound library of 2 million, two of which ultimately demonstrated clinical improvement in animal disease models. The total time from project launch to preclinical testing was four months.
Although experimental validation in experimental systems is still necessary to eliminate false leads from in silico experiments, data-driven drug discovery is expected to improve drug development programs by lowering prices and driving innovation. Several conditions must be met to enable data-driven drug discovery:
-
Unbiased data. Genomics-driven drug discovery based on genomewide association studies (GWAS) could streamline the drug discovery pipeline (e.g., the association between a loss-of-function variant of PSCK9 and low-density lipoprotein cholesterol led to the successful development of PSCK9 inhibitors to lower cholesterol). However, a continued lack of diversity in many GWAS cohorts is a problem as it may lead to spurious disease associations while leaving out large groups of patients who still need treatment.
-
Diverse data types. Integrating various data sources from omics data, electronic health or medical records, and wearables presents a logistical challenge to researchers who need to make sense of complex information from various sources to draw meaningful conclusions.
-
Data sharing. Data sharing and collaboration can expand our understanding of human disease. Still, concerns about privacy and protecting intellectual property are reasons researchers hesitate to share data.
Lifebit supports the life sciences sector in accelerating Drug Discovery 2.0 through these three key elements:
-
Multi-modal federated data: Managing, linking, and extracting insights from diverse data types across various sources and modalities (e.g., clinical, molecular, imaging). Implementing a democratized, user-friendly, no-code point-and-click solution tailored to drug discovery researchers for swift and accurate insights in days or weeks.
-
Data standardization: Seamless harmonization through automated tools to accelerate research capabilities.
-
End-to-end analytical solutions: Moving away from isolated point solutions to provide comprehensive disease insights, ultimately aiding in target identification and verification.
Figure 2. How Lifebit supports the life sciences sector in accelerating Drug Discovery 2.0.
Summary
Despite false leads and failed drug development programs, the next phase of drug discovery is on the horizon. Powered by high-powered computational methods relying on large-scale datasets, Drug Discovery 2.0 is expected to bring novel drugs to market faster and decrease burdens on health systems and patients everywhere. To facilitate the use of these technologies, massive, secure, transformed, standardized, and high-quality data will be needed.
About Lifebit
Lifebit provides federated data analysis services for clients, including Genomics England, Boehringer Ingelheim, Flatiron Health and more, to help researchers transform data into discoveries.
Interested in learning more about how we accelerate research insights in drug discovery?
Featured news and events
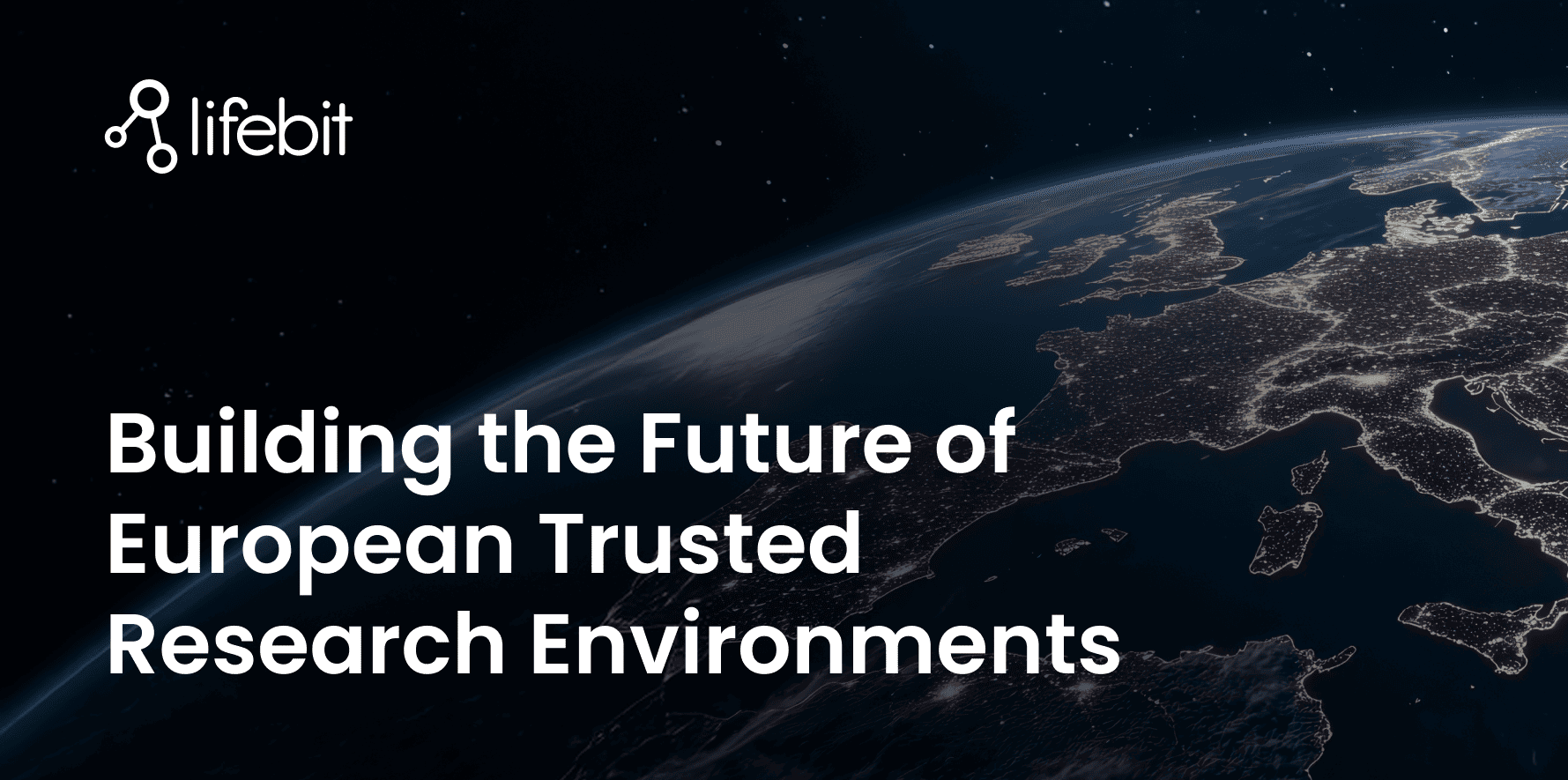
2025-03-26 11:17:46
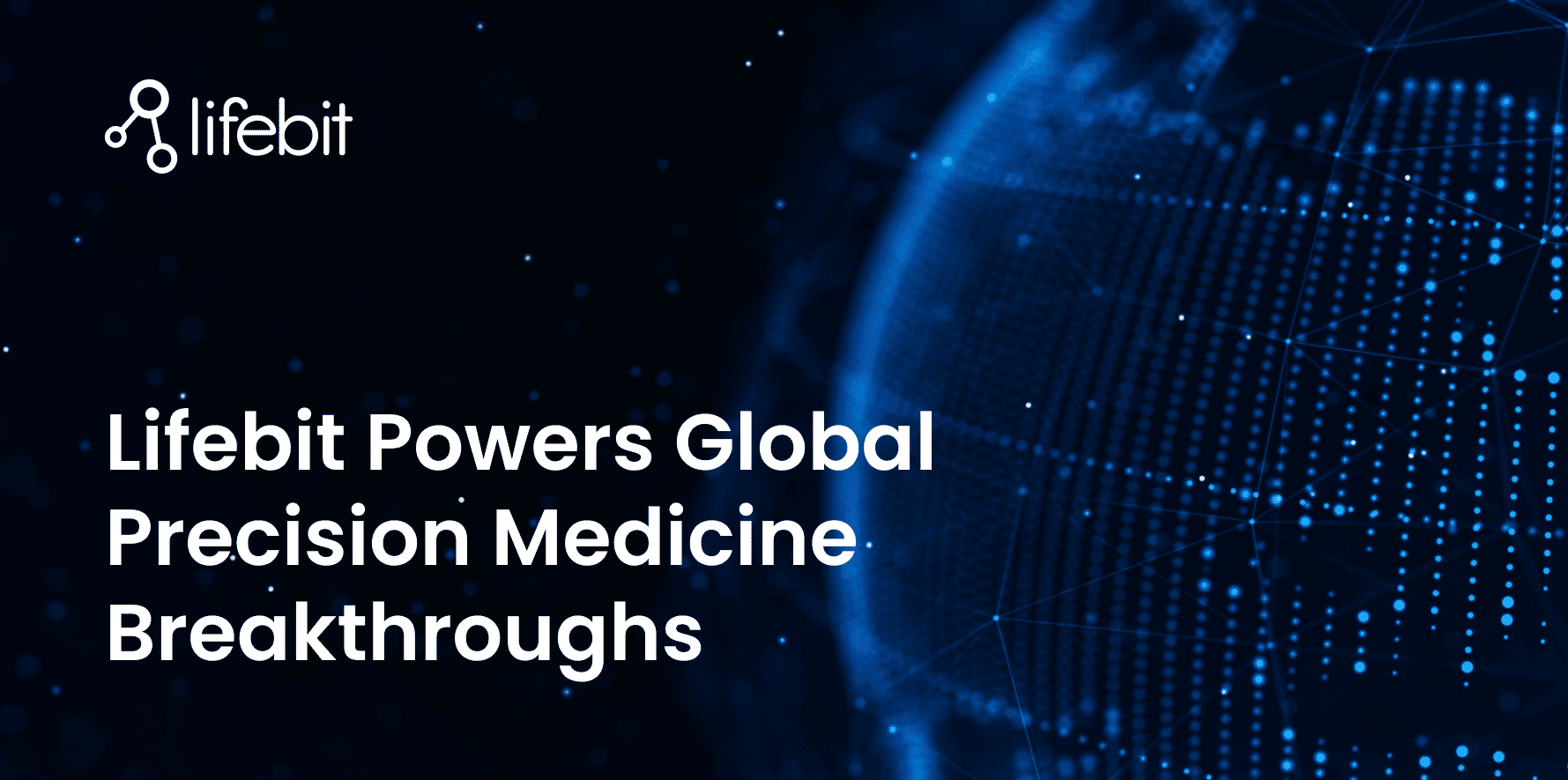
2025-03-14 15:45:18
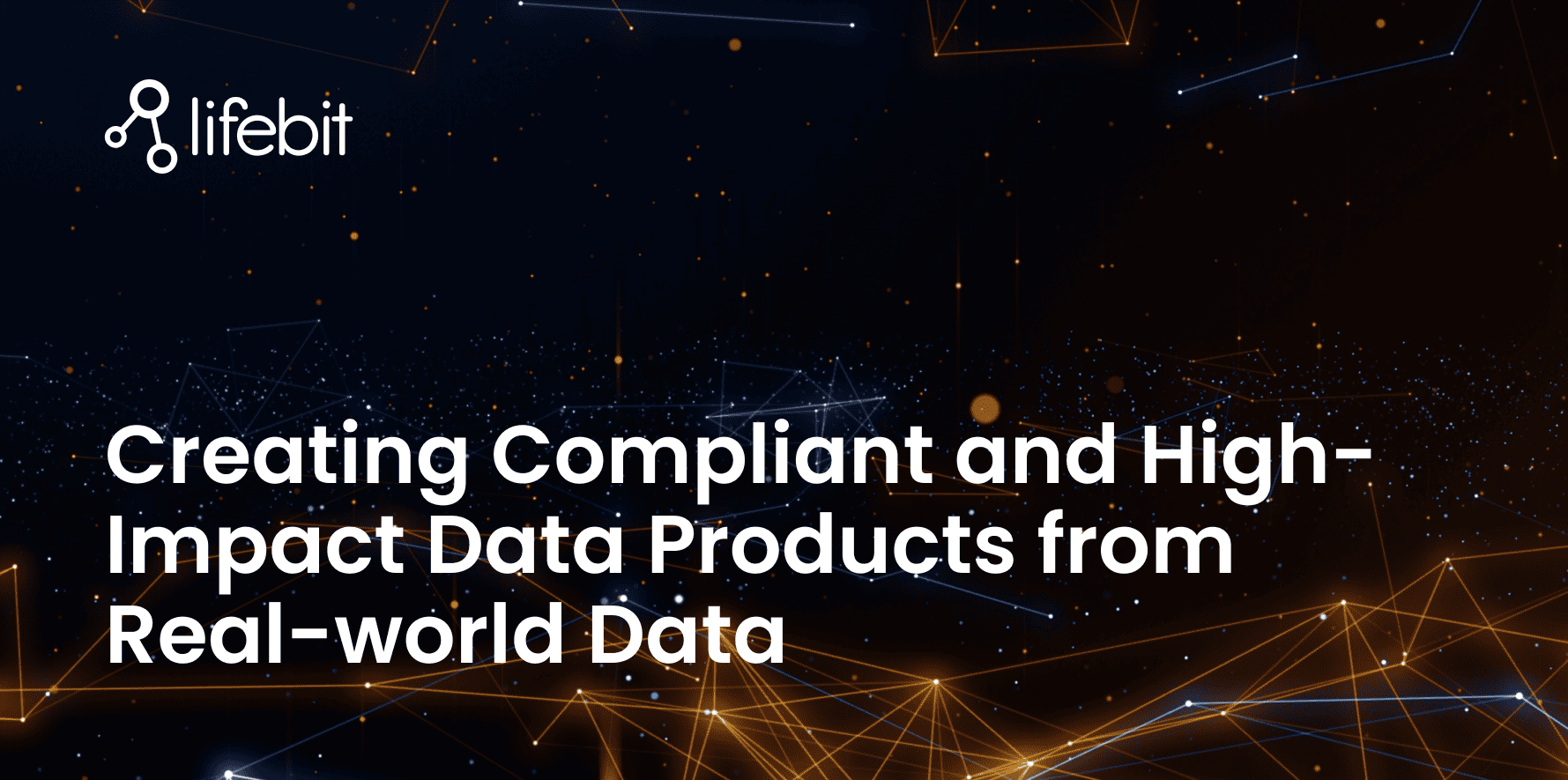
2025-03-05 12:49:53
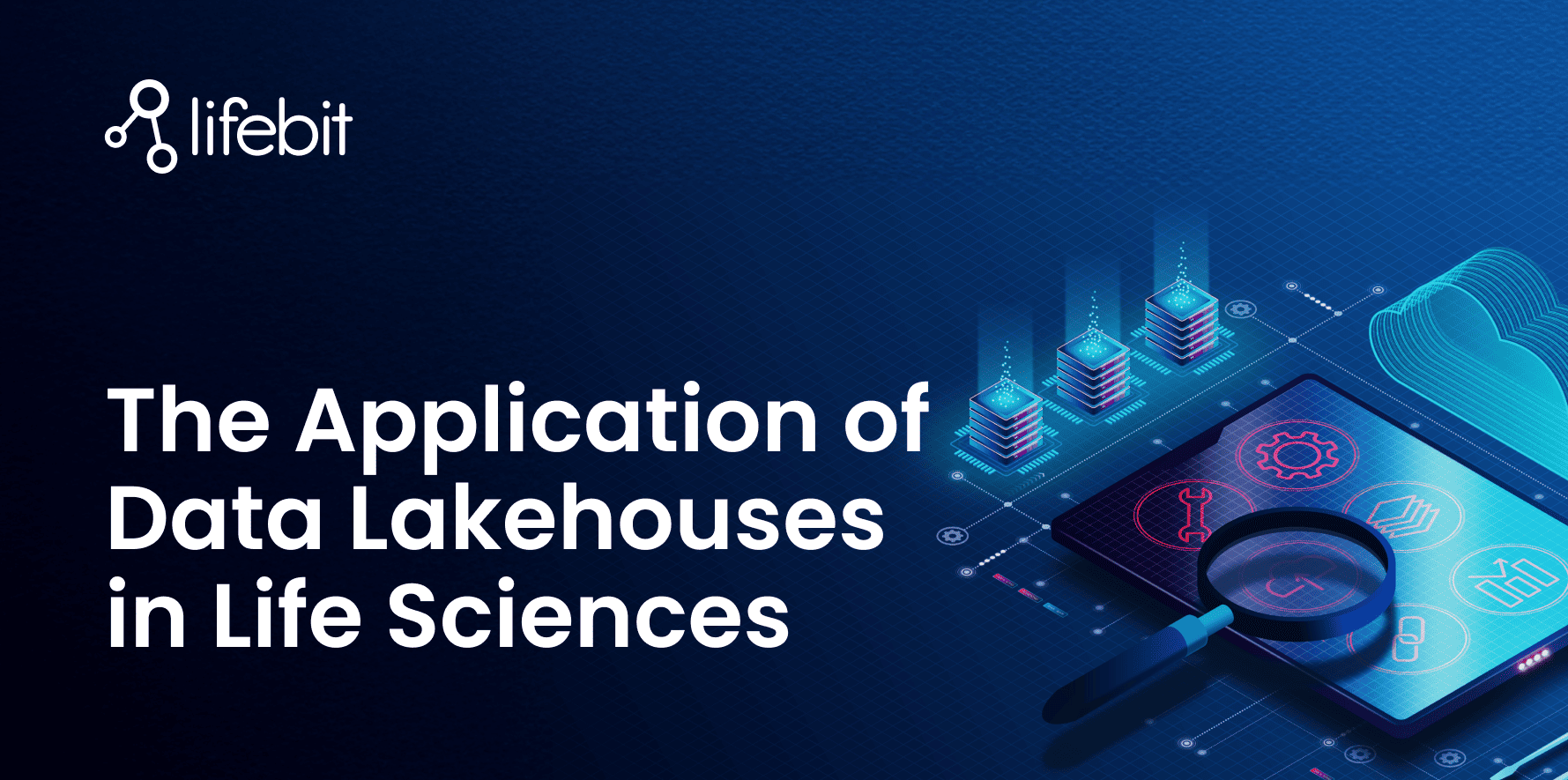
2025-02-27 10:00:00
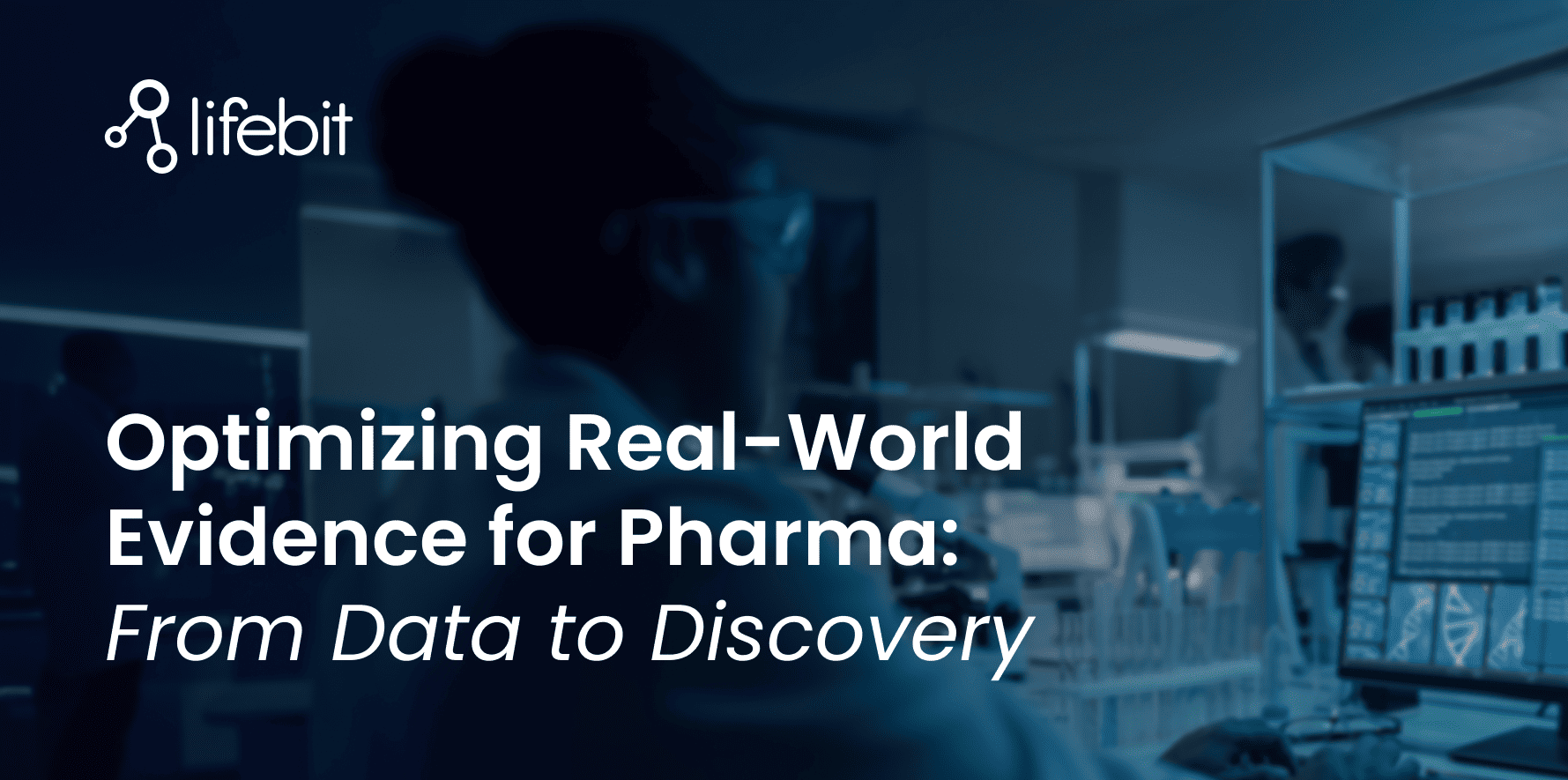
2025-02-19 13:30:24
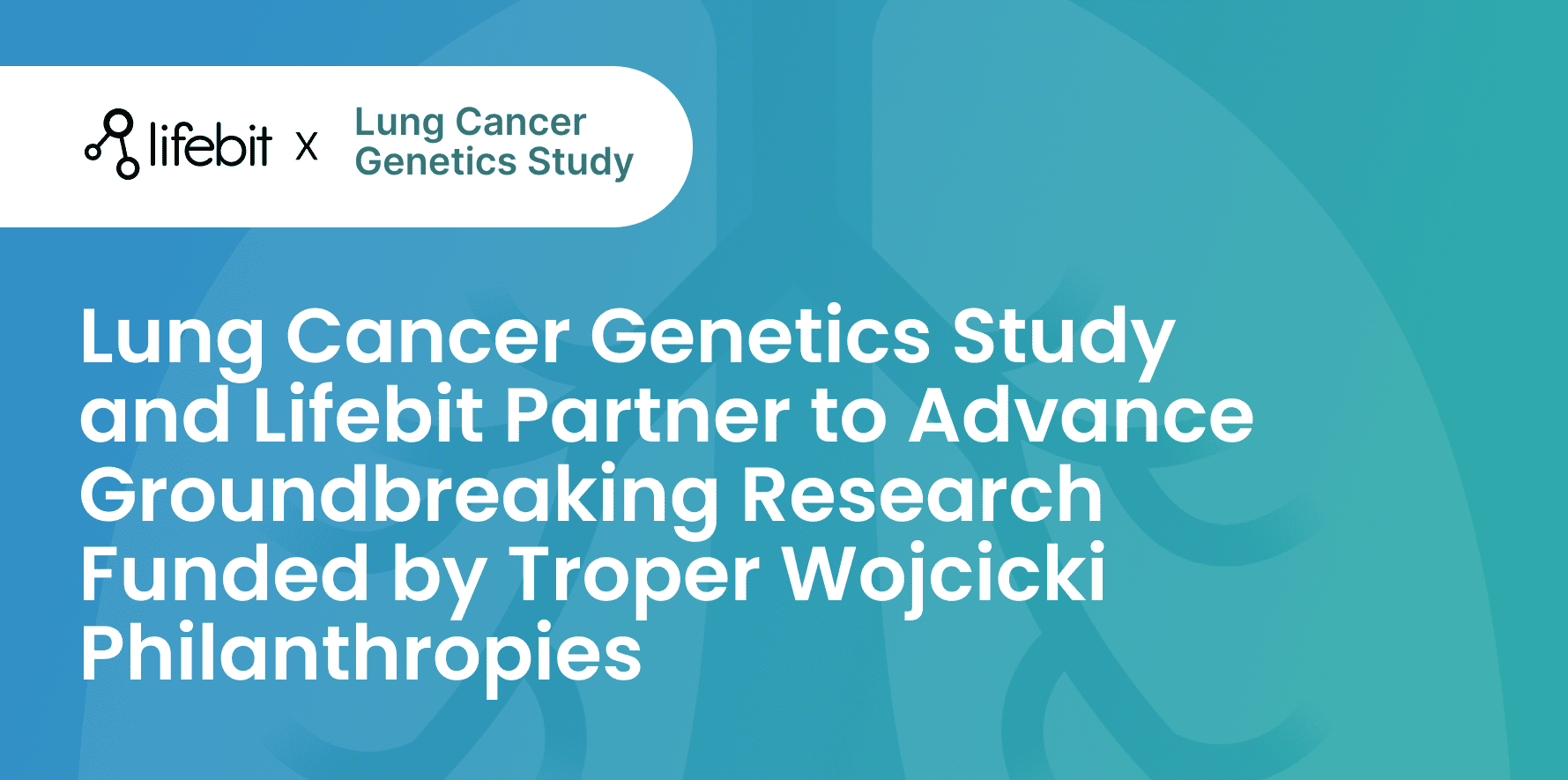
2025-02-11 08:39:49
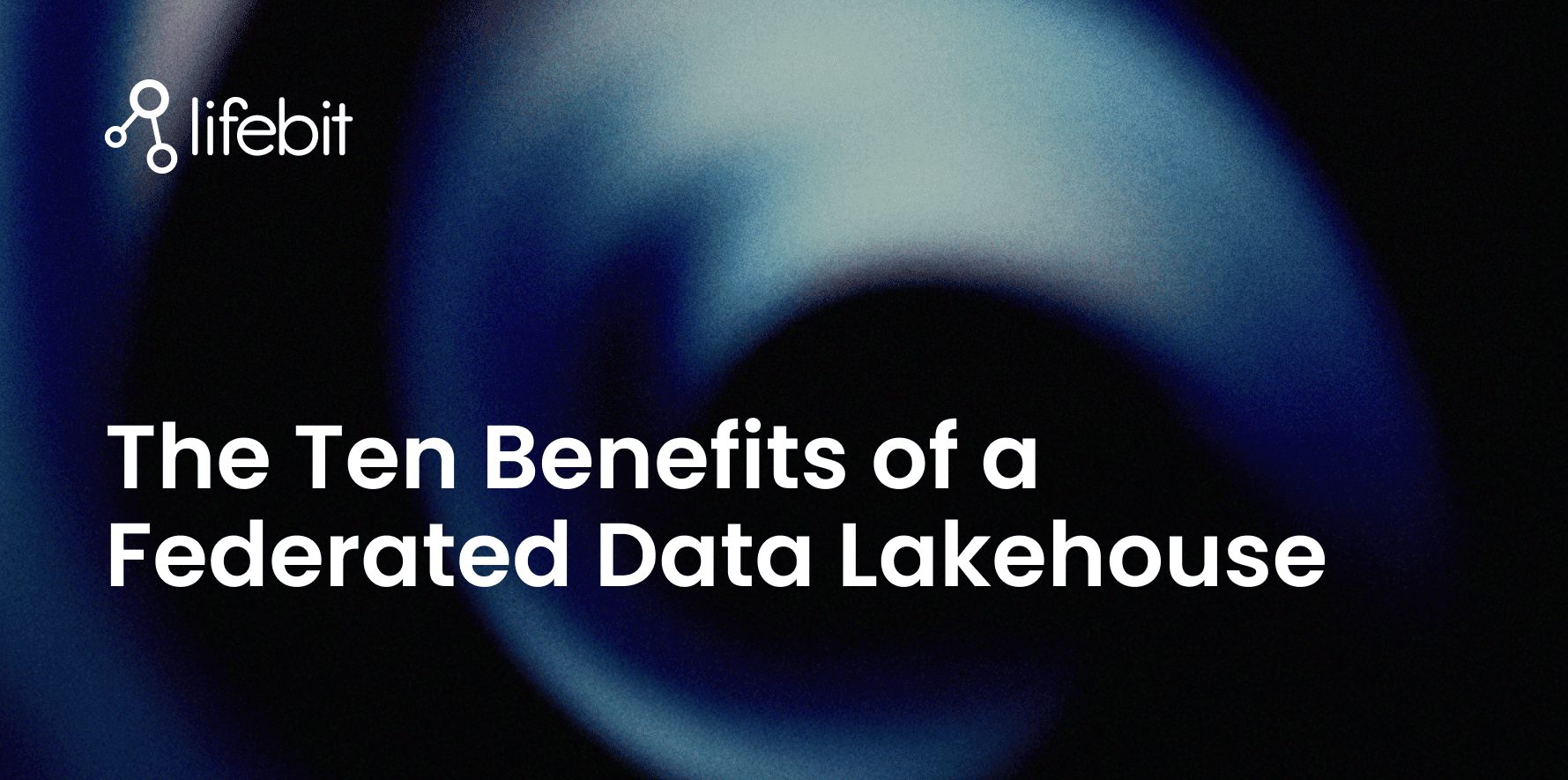
2025-01-30 12:47:38
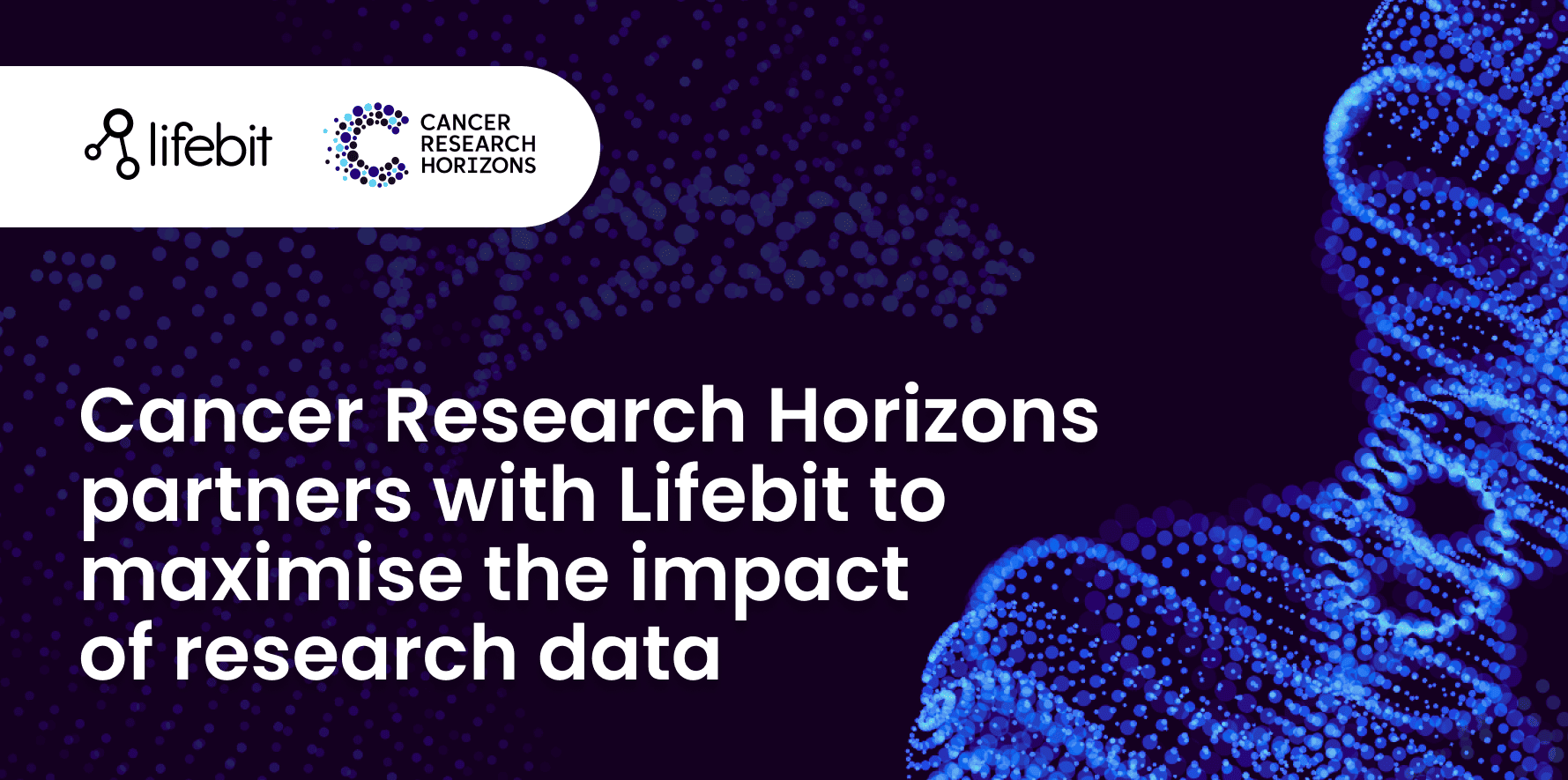
2025-01-28 08:00:00
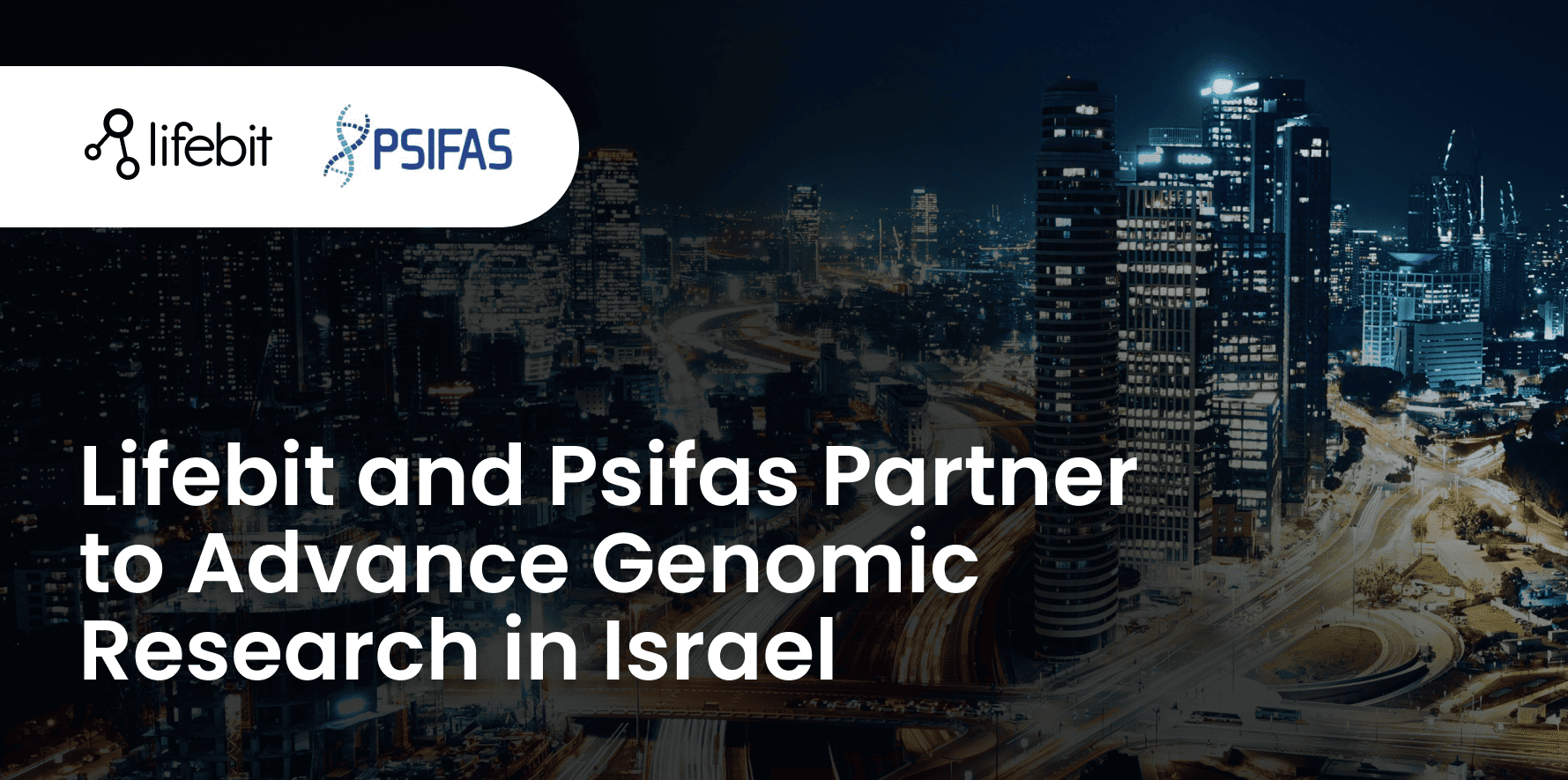
2025-01-23 09:07:20

2025-01-08 13:58:41