
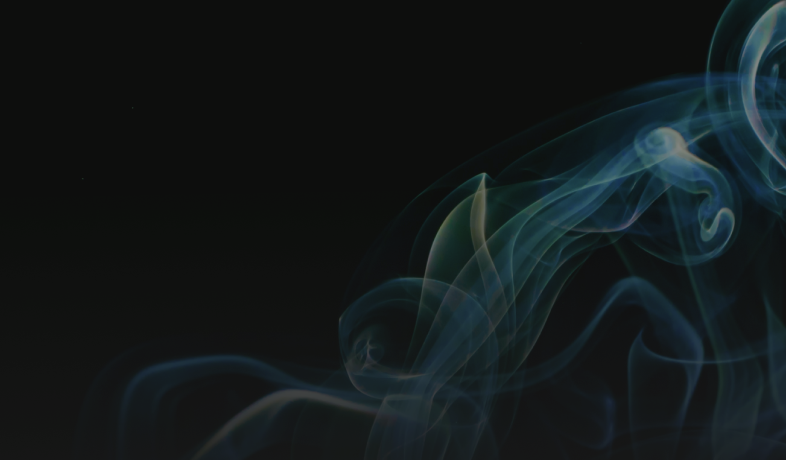
Finding Genetic Variations Associated to Complex Diseases: Needle in Haystack
Lifebit
There are 3,000,000,000 base pairs in the human genome, which define our individual code. 99% of these are identical for all humans- only the remaining 1% can help explain our differences. Finding these differences, or single nucleotide polymorphisms (SNPs), is the equivalent of looking for a needle in a haystack. SNPs are random and can be harmless, or they can be correlated with specific diseases or traits.
Genome-wide association studies (GWAS) have gained traction since the mid 2000s, with over 7,800 GWAS studies performed which have facilitated the detection of over 159,200 unique ‘needles’ – SNP-trait associations, all archived in EMBL-EBI’s GWAS Catalogue.
The basic premise behind GWAS is to compare the genetic variations between two cohorts: the first is a set of individuals with a specific disease or trait being studied, and the second is the control group. Essentially, researchers are able to identify genetic variations associated with a particular disease.
Phenome-wide association studies (PheWAS), are the complementary ‘inverse’ approach to GWAS, and examine many different phenotypes to see which are associated to a given genetic variant. The fundamental difference between both methods is the direction of inference – in GWAS it is from outcome to exposure, while in PheWAS it is from exposure to outcome.
GWAS enabled a major shift in the way we link genotype to phenotype & map disease traits
GWAS and PheWAS have proven to be the most powerful ways of revealing the effects between genetic variations and phenotypic outcomes. Before such methods were implemented, researchers had relatively low-resolution approaches (i.e. linkage mapping) or more targeted approaches where candidate genes were resequenced in cohorts of interest. Post-GWAS is an entirely different story – we now have a high-resolution and unbiased approach to unearthing regions or genes that may have not previously been on our radar.

GWAS and PheWAS analyses are performed for the following three main purposes:
- Providing potential targets for therapy by highlighting underlying molecular pathways,
- Identifying markers used to predict individual disease risk or phenotypic trait (Polygenic Risk Score estimations), and
- Cohort analysis for better patient stratification and clinical trials.
GWAS have successfully contributed to our understanding of disease mechanisms, with the GWAS poster child being age-related macular degeneration (AMD), the leading cause of irreversible vision loss in individuals over the age of 60. GWAS has unambiguously revealed that the complement pathway is involved, which was not previously known to play a role in the disease. There are many other exemplars of GWAS successes, including type 2 diabetes, schizophrenia and auto-immune diseases.
GWAS results are highly reproducible and extremely powerful as they enable valid predictions, or polygenic risk scores (PRS), in new unexplored datasets. PRS allow the stratification of a population based on the sum of trait-associated SNPs weighted by their effect sizes. Essentially, such risk scores provide an overall measure of an individual’s genetic liability to develop disease – regarded by many as the holy grail. Large-scale initiatives, such as the UK 100,000 Genomes Project, are ideal databases for GWAS/PheWAS analysis and determining PRS – the bigger the dataset, the more variant-trait associations can be identified.
GWAS and PheWAS analysis challenges – the criticism & controversy
GWAS and PheWAS have allowed the broad characterisation of the genetic basis of traits and diseases, however, several challenges remain. Let’s address the elephant(s) in the room.
Extrapolating findings to other populations
Although GWAS and PheWAS studies has been revelatory in many aspects, they are still predominantly focused on European populations (88% of studies in 2017) with 72% of discoveries from participants recruited from three countries (US, UK, Iceland).
This is especially concerning for the translatability of polygenic predictions from one population to another. If researchers were to develop a polygenic risk score for having a heart attack, for instance, the scores would be meaningless if applied to any other population besides white Europeans. If GWAS is to be a truly equitable and useful tool to predict disease risk, studies need to be repeated in more diverse populations.
Recently, there has been a significant push to include other ethnic groups and admixed populations as there is a pressing need to extrapolate findings to non-European populations and to increase the statistical power of these studies.
Furthermore, the lack of diversity should raise red flags, especially when performing GWAS and PheWAS analyses to improve patient stratification for clinical trials. Since these analyses can be performed in cohorts as small as 250 individuals (depending on the number of SNPs tested and effect size), findings may not be easily translatable to other patient groups, as ancestry admixture plays a significant role.
Scaling analysis to accommodate growing cohort sizes
Besides genetic diversity and careful cohort selection being recognised challenges in the field, the importance of cohort sizes has become a critical factor in assuring the statistical power of findings.
Early GWAS and PheWAS studies did not reveal many correlations mainly due to the small cohort sizes. Smaller sample sizes do not generate enough statistical power to find associations linking markers and phenotypes (this also depends a lot on the genetic architecture of the trait(s) you are focusing on – oligogenic vs polygenic).
But as public and private datasets grow in size and complexity (i.e. UK Biobank, or even commercial databases such as 23&Me), researchers now have access to treasure troves of genetic data, allowing them to enhance the quality of their cohorts, and by default the significance of their results. Case in point – a recent GWAS study published in Nature Genetics used data from 1.1 million individuals to assess their adventurousness and willingness to take risks.
However, as GWAS and PheWAS continue to evolve in complexity and more data is being analysed, we now face the issue of scaling analysis. Specifically, when we’re now talking about hundreds of thousands to millions of individuals in a single study, we need to take into account how computationally challenging and expensive that can turn out to be.
Uniting disconnected data
Often times, high quality GWAS and PheWAS studies grow beyond the capacity of a single institution- which is surely even more the case now considering the 1M+ samples sizes. This requires researchers to unite disconnected data pulled from different public and/or private data repositories.
Uniting sensitive and disconnected data, however, can prove challenging and a maximum security risk if you have to physically transfer or share it. Researchers need a practical and efficient way to do so, without having to put at risk their data… or their sanity.
Overcoming GWAS & PheWAS challenges by getting analysis on-demand.
In an ideal world of GWAS and PheWAS analyses, cohorts would include a large number of individuals, datasets would be diverse in terms of ethnicity and the genetic and phenotypic data would be centralised in one big public database. Effectively, this would eliminate computational issues and researchers could easily run and scale the analysis on-demand in the fastest, most scalable and cost-effective way.
One can always dream, right?
Well, there are specific ways for researchers to overcome challenges associated with GWAS and PheWAS analyses.
To accommodate for large cohorts, researchers should turn to the cloud for infinitely scalable compute resources. At Lifebit, we have developed cloud-native optimised implementations of both GWAS and PheWAS workflows, which are freely and accessible on-demand in the Lifebit CloudOS platform. These state-of-the-art standardised and reproducible pipelines harness the power of the cloud for elastic resource provisioning, while at the same time, keeping costs low (> 80% cloud cost reductions) with cost saving instances and deployment efficiency.
Besides improving scalability and reining in costs, researchers should be able to perform GWAS and PheWAS analyses on distributed data from private and public datasets. At Lifebit, we have created the only federated data analysis platform – the Lifebit CloudOS platform – which allows researchers to access disconnected data without having to deal with the inefficiencies of transferring or copying large volumes of data. This provides an ideal foundation for a globally fragmented and distributed GWAS community which stores data in countless isolated databases around the world.
By using Lifebit CloudOS technology for your GWAS and PheWAS analyses, you will be able to:
- Infinitely scale your GWAS & PheWAS analysis to study ever-increasing cohort sizes
- Access public data and combine it with your private data for federated analysis, allowing you to cover more ground without having to transfer massive datasets
- 100% reproducible, compliant and FAIR GWAS and PheWAS pipelines – optimised for cloud-native usage that ensures maximum scale, speed and cost-minimisation
- Minimise and monitor costs and runtimes, ensuring you stay within your research budget, and
- Provide intuitive visualisation of your GWAS results
By robustly developing end-to-end GWAS and PheWAS workflows, researchers will be able to avoid ad-hoc analyses, and embrace scalable and reproducible bioinformatics workflows in production with the CloudOS platform.
Try it!
If you are curious to see what our GWAS and PheWAS workflows are capable of achieving, check out the ones we have already run through our public jobs links: GWAS & PheWAS.
Have your own data to test drive? Run our scalable GWAS and PheWAS workflows on the CloudOS platform.
If you are already using Lifebit’s GWAS & PheWAS pipelines on CloudOS, we would love to know what you think! If you’re interested in running our pipelines, contact our Customer Success team below, they would love to help you out!
Featured news and events
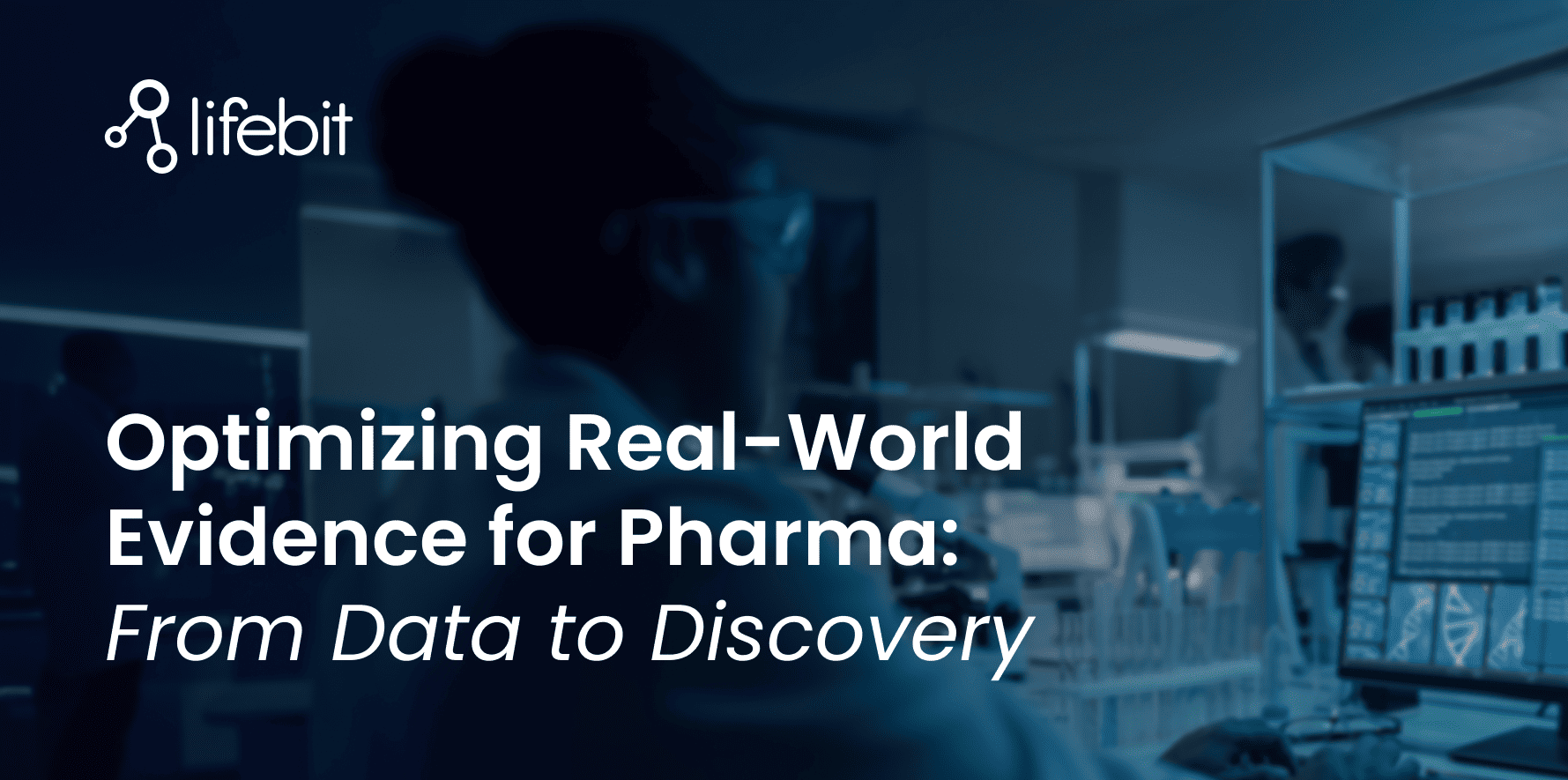
2025-02-19 13:30:24
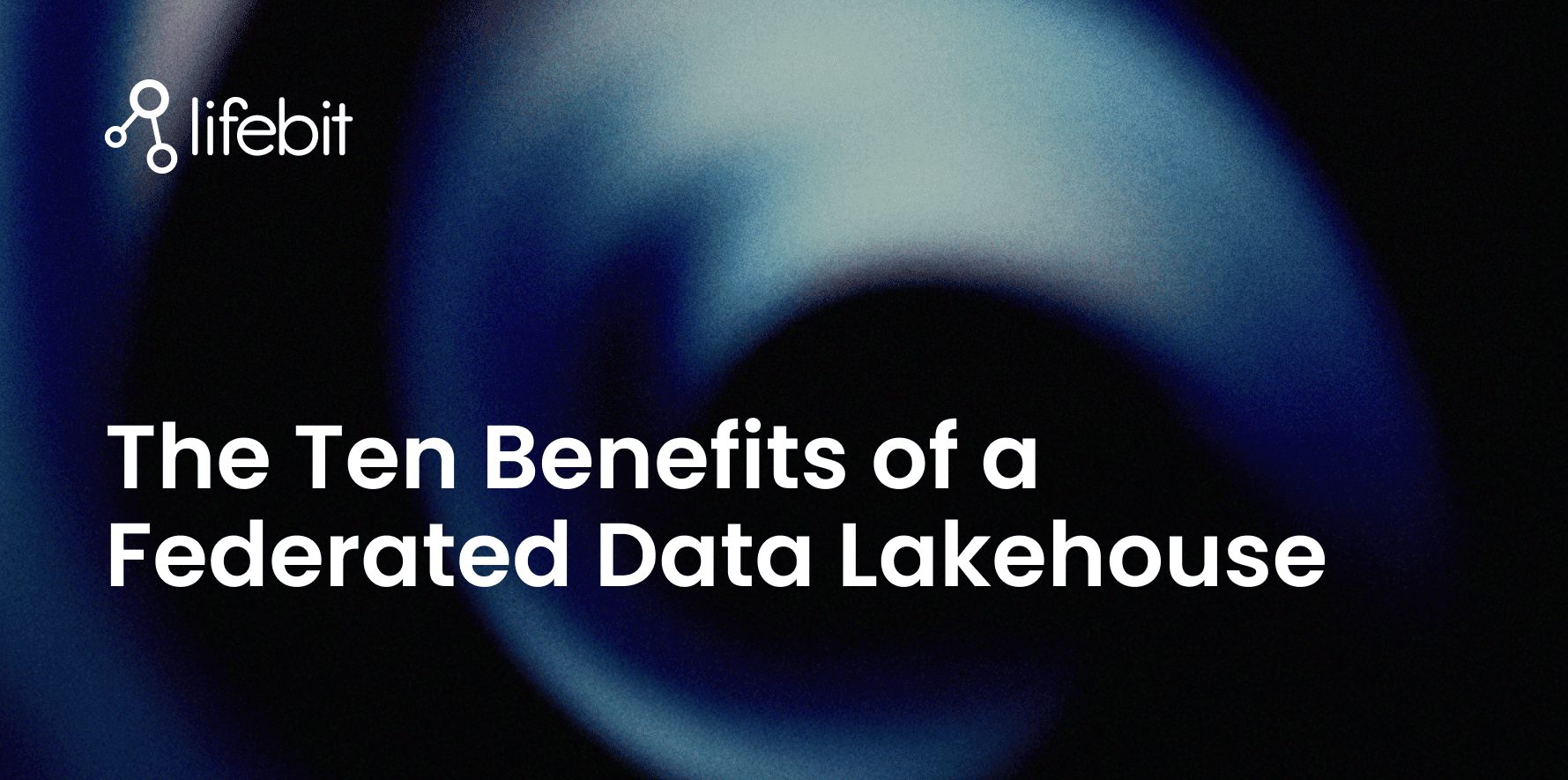
2025-01-30 12:47:38
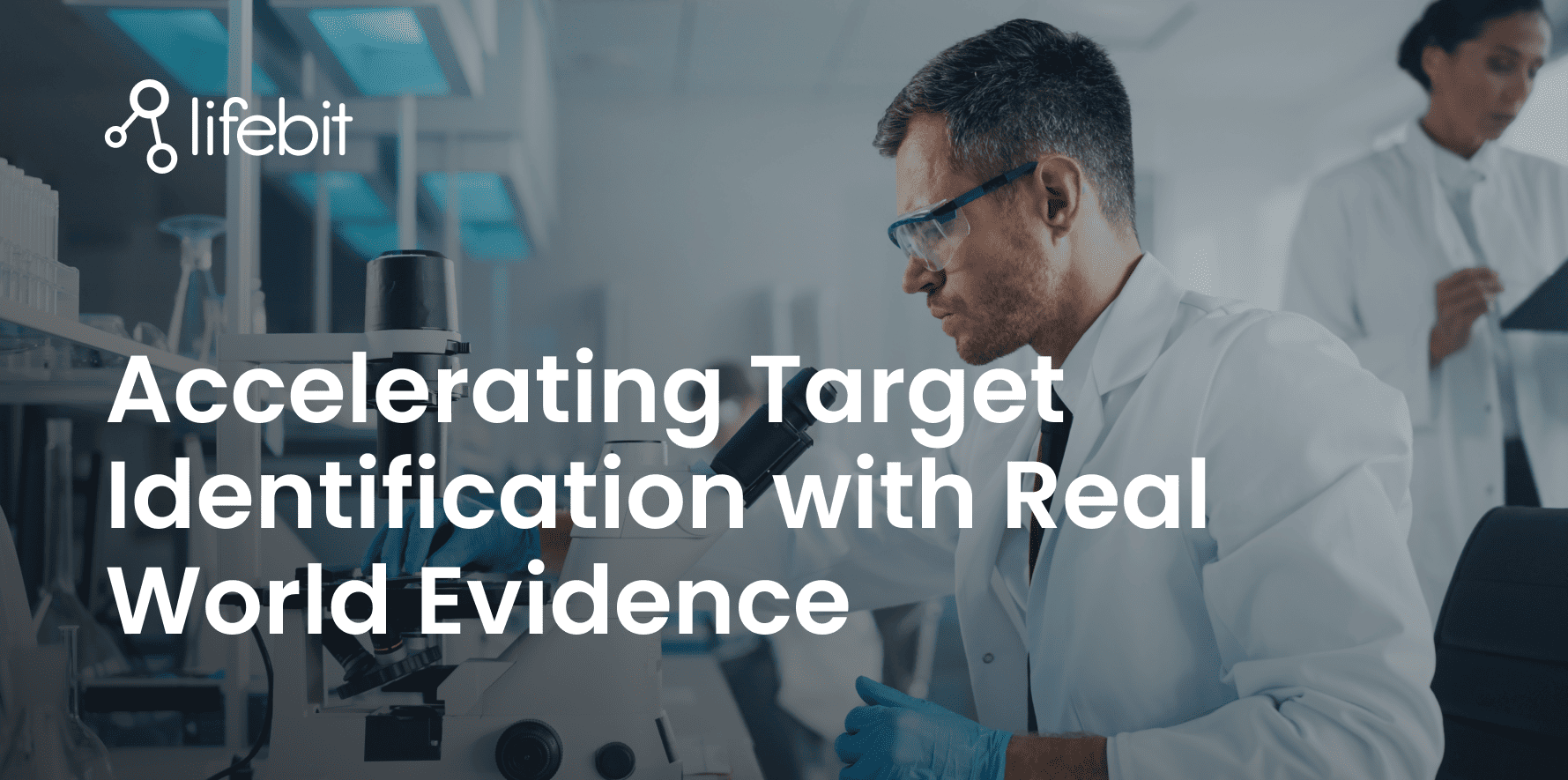
2024-12-06 15:53:10
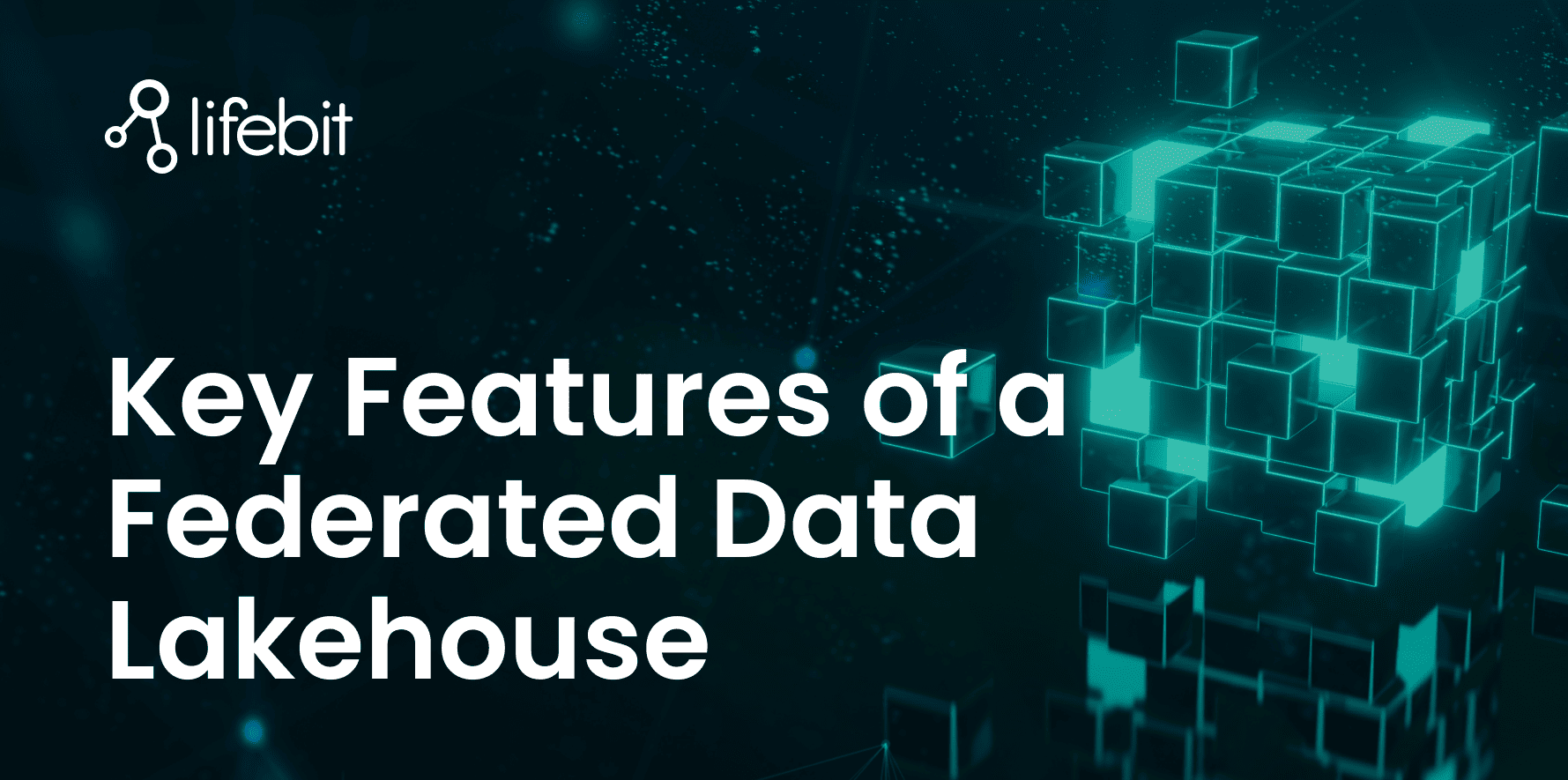
2024-11-27 12:35:34
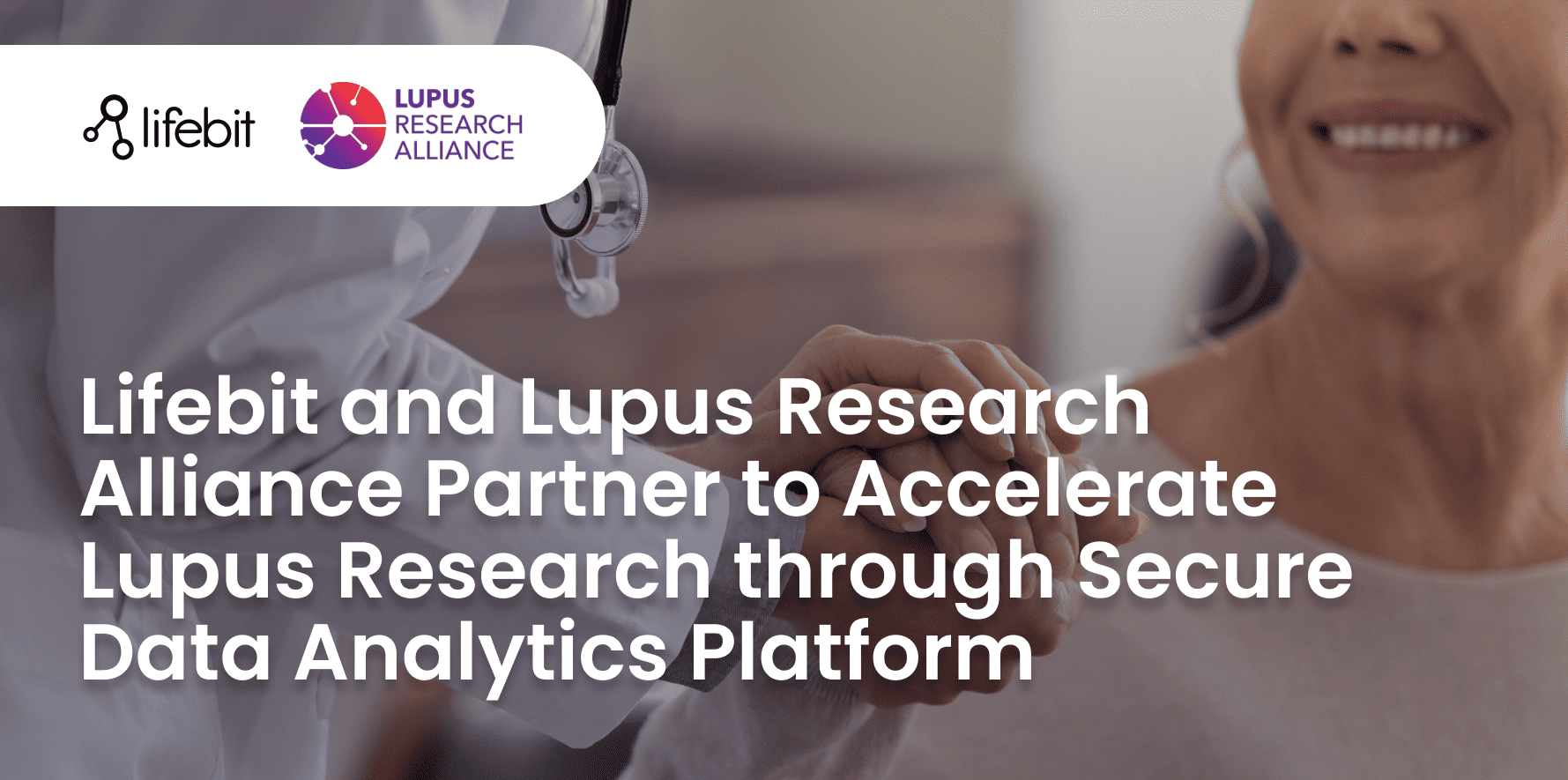
2024-11-27 09:15:04
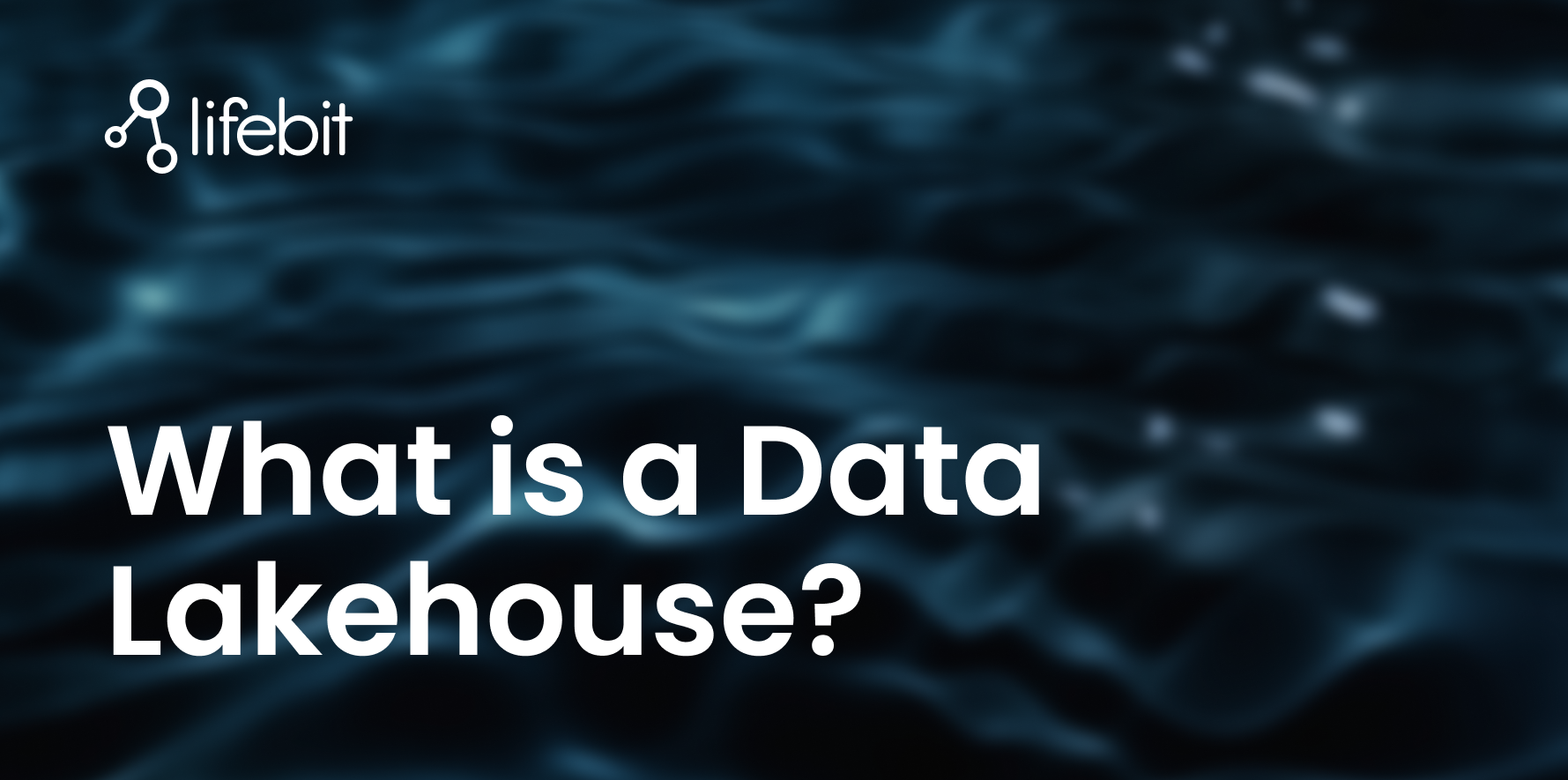
2024-11-20 09:41:45